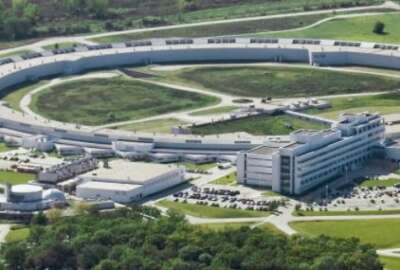
Hubbard Radio Washington DC, LLC. All rights reserved. This website is not intended for users located within the European Economic Area.
Hubbard Radio Washington DC, LLC. All rights reserved. This website is not intended for users located within the European Economic Area.
With more on this project, Argonne computer science leader Prasanna Balaprakash joined Federal Drive with Tom Temin.
Best listening experience is on Chrome, Firefox or Safari. Subscribe to Federal Drive’s daily audio interviews on Apple Podcasts or PodcastOne.
It didn’t take long after the advent of the automobile for traffic jams to become a dismal feature of modern life. Now researchers at the Argonne National Laboratory are working to discover a way to model and forecast traffic so it can be mitigated by re-routing. With more on this project, Argonne computer science leader Prasanna Balaprakash joined Federal Drive with Tom Temin.
Interview transcript:
Tom Temin: Dr. Balaprakash, thanks for joining me.
Prasanna Balaprakash: Thanks for having me.
Tom Temin: Now, this is a problem that people have tried to attack for many, many years: How to mitigate traffic. And I guess my understanding of traffic is that it is a fluid and needs to be looked at through the eyes of fluid dynamics. Am I all wet? Or is that really the case?
Prasanna Balaprakash: You are very close. There are different ways to model traffic and modeling traffic with the fluid flow is definitely one of the modeling methodologies that is very close to the underlying physics. So the approach that we took is also pretty close to the idea of fluid flow. It’s called diffusion process. So basically, the diffusion process is something that is used to model particles that move from higher concentration to low concentration, which is pretty close to what you would expect with the traffic.
Tom Temin: And of course, people have been trying to solve this for a long time; what new tools do we have? I’m guessing they’re in the area of predictive analysis and artificial intelligence. But what are the new tools we have to take on this old problem?
Prasanna Balaprakash: Okay, so that’s a fantastic question. So let me take a step back. The problem of traffic forecasting is very, very complicated, it has a lot of local interactions. And the fact that something happens far away can affect the other regions of the traffic network, and so on, so forth. So the problem is very complex, there are a lot of intricacies and modeling this mathematically has been a challenge. So people reduce that to make assumptions and reduce the problem and try to develop mathematical models that can provide forecasts. But the challenge is all these assumptions leads to reduction in predictive accuracy. So that has been a challenge so far. With the recent tools in particular, we understand the traffic network infrastructure people, they collect a lot of data, starting from the sensors on the road all the way to using traffic trajectories from cell phones, in an anonymized way, and so on, so forth. So now we have lots and lots of data to play with. And at the same time, there are advances in artificial intelligence, and particularly a class of approach called machine learning. It’s a purely data driven approach, instead of doing things more in a mathematically precise way. Now, you have these learning approaches where you throw in tons of data. And these approaches learn from the data and produce a model, which doesn’t require precise mathematical definitions or mathematical modeling. But it can give much more precise and much more predictive accuracy, then the simplified models.
Tom Temin: Because I think there would be another big variable in here that may not apply in other endeavors, other areas of looking at dynamics, and that is the human factor, which is maybe the most unpredictable part of people inside the car, the nut behind the wheel, so to speak.
Prasanna Balaprakash: Yeah, totally. So that’s why modeling the human in a sense, like what is an individual is going to do, is very hard. And capturing that in a mathematically precise way is very, very hard. So that’s why this data driven approach looks at general trends, and also looks at the correlations based on the day of the week, a month of the year, seasonality, and even such as, say, some sports related events is happening, and there is traffic. So all these we don’t need to write precise models for each and everything. Instead of that we have data and we let the data speak. And we want to learn these correlations automatically. And use this information in a model that can give us much better predictive accuracy.
Tom Temin: We’re speaking with Dr. Prasanna Balaprakash, computer science leader at the Argonne National Laboratory. Well, tell us about the program itself. Where did you get the data sets that you are using? Where did you load them into? And what’s the status of it at this point?
Prasanna Balaprakash: So the data that we are working on is from California highway network systems. And there is a dedicated infrastructure that collects lots of data from the whole of California. In particular, we were looking at Los Angeles, which is prone to traffic jams and a lot of traffic issues.
Tom Temin: Yeah, I’ve been there, I’ve noticed.
Prasanna Balaprakash: Okay, so that’s of special interest. But we are looking at data in a much bigger scale, throughout the whole California traffic network. So there is a system called PANS, which collects the data throughout the network, and one can take the data and analyze the data. So that’s where we started. So we worked with around 11 thousand sensors, which are sort of spread throughout California. We downloaded around one year worth of data and developed these predictive models.
Tom Temin: Alright, and have you learned anything at this point?
Prasanna Balaprakash: Many things. The first and foremost: The data is noisy. And we have to do a lot of pre-processing to make sure that the data is in a form that we can use. And we can inject into our machine learning models. So there are a lot of failures in the sensors, because the sensors are prone to these type of failures. And because of the weather, because of various types of external conditions. So these are the sensors that stay out in the road. So we have to deal with the noise, we have to deal with the failures. And we have to do pre-processing to make sure that the data is in proper form. So once we have the data, then the next thing is the volume of the data. So it’s both a blessing and also a disadvantage. So we have a lot of data, we have large volumes of data and processing this data is hard and presents numerous computing challenges. So that’s where the supercomputing capabilities at Argonne comes in. So the algorithms are part of the puzzle, the data is a major chunk of the puzzle. But the ability to process this data, to learn from this data, using large supercomputers is a critical part of the puzzle. So if you take the data, and if you try to do this, try to build a predictive model on a single computer, it might take a week, more than a week, full time. And that’s something that we cannot afford. So what we did is try to scale these approaches on large numbers of compute nodes, we call them in a supercomputer. So supercomputers, think of this as a number of individual compute nodes put together. So we scale them on these big machines, so that we can reduce the training time or the analysis time from a week to less than three hours. So now we can process this massive data within three hours. We can build predictive models within three hours. And this is a game changing capability for traffic management scenarios. Because the ability to process the data in three hours, you can do that overnight and build a predictive model and deploy the next morning. So your model, you can keep on updating this model within a very short time. And as you mentioned, these type of models can by sheer nature of being updated more frequently provide much better accuracy than otherwise.
Tom Temin: Well, once you have these models, and you can do predictive analysis of the traffic, what’s the practical application? Because you can admire the problem, but can you do anything about it?
Prasanna Balaprakash: Your ability to predict traffic in advance and in particular the ability to predict traffic conditions as soon as some events happen in a traffic network, provide numerous advantages for proactive traffic management. You can start rerouting traffic in a much better way, in a proactive way, as opposed to a reactive way. You don’t wait until something happens. But you see that there is something wrong that is happening. So for example, you have a predictive model, and you are looking at the trend from the predictive model and the actual traffic, you are monitoring these differences and say, ‘something is wrong on this particular network.’ And as soon as you see those kind of events, or those kind of discrepancies, you can start rerouting traffic in a much better way. So ability to predict traffic incidents and traffic conditions, 30 minutes before it gets really, really worse, makes a big difference. So those type of capabilities are something that the traffic management centers are looking for. And these type of approaches are quite effective and useful for those problems.
Tom Temin: Now, this output is being generated by the Energy Department. Will you share it with the Transportation Department? What becomes of what it is that you learn in this project?
Prasanna Balaprakash: Okay, so the Department of Energy. So this is funded by Department of Energy within that specific division called VTO: Vehicle Technology Office. And one of the overarching goal is to address the energy challenge. So we are also looking at this problem from the energy perspective, meaning fuel efficiency, people productivity, and so on, so forth. So that’s why this is a model. If we manage to address these type of problems, then it can enable other type of capabilities. So there are talks with the traffic management centers, we are in touch with them at this point. But this is a work in progress. And we are just scratching the tip of the iceberg. So the next thing is in how this thing will work in production, we have to do the simulation, we cannot just take this and put this right now, because we have to do all the testing beforehand. So we had to do a simulation. So that’s the other piece of the project, which is led by LBNL, Lawrence Berkeley National Lab in Berkeley. So they will use these type of models within simulation and try to see what are the different types of practical strategies that one could devise that could help address this problem. So it’s a number of steps that we have to take to make sure everything works, and then start thinking about the deployment.
Tom Temin: Dr. Prasanna Balaprakash is computer science leader at the Energy Department’s Argonne National Lab. Thanks so much for joining me.
Prasanna Balaprakash: Thanks, Tom. Thanks for having me.
Copyright © 2024 Federal News Network. All rights reserved. This website is not intended for users located within the European Economic Area.
Tom Temin is host of the Federal Drive and has been providing insight on federal technology and management issues for more than 30 years.
Follow @tteminWFED