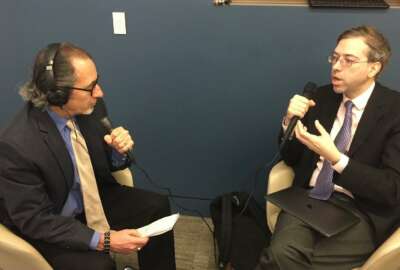
Artificial intelligence is coming to the way the National Oceanic and Atmospheric Administration monitors populations of endangered species.
Best listening experience is on Chrome, Firefox or Safari. Subscribe to Federal Drive’s daily audio interviews on Apple Podcasts or PodcastOne.
Artificial intelligence is coming to the way the National Oceanic and Atmospheric Administration monitors populations of endangered species in the polar regions, including, beluga whales, ice seals and polar bears. They do it from a camera-equipped, turboprop airplane. How they came to partner with Microsoft is a story in itself. For more, Federal Drive with Tom Temin heard from Microsoft principle scientist with the AI for Earth program, Dan Morris.
Interview transcript:
Tom Temin: Dan, good to have you on the show.
Dan Morris: Good to be here.
Tom Temin; So tell us about the AI for Earth program itself at Microsoft. This is something you work with I guess a lot of entities doing this type of research.
Dan Morris: That’s right. So AI for Earth drives innovation at the intersection of artificial intelligence and environmental sustainability. We do that through grants, and we also do that through technology development, in close partnership with organizations like NOAA, working on problems in environmental science that we think could be dramatically accelerated with Cloud and AI tools.
Tom Temin: And how did you come to partner with the NOAA group?
Dan Morris: This particular collaboration has an interesting round about story. A colleague of mine at Microsoft happened to serve on jury duty with one of the scientists at NOAA. They got to talking, she said I got two million pictures of seals and it’s driving me crazy, and my colleague at Microsoft thought, I bet that we can help make your life a little easier with AI. That led to a project a couple of years ago at what we call our one week hackathon at Microsoft, which is a big summer event where employees basically take a couple days off from their day jobs to work on something they’re passionate about. Many folks do work on problems related to environmental science during that time. Then we kind of built some initial prototypes to help NOAA scientists use AI to find seals in those millions of images that they’re capturing. That really kicked off a project that’s been running through today.
Tom Temin: So they would fly over the territory that they were trying to count the seals in and get back pictures. From what I looked at, a couple of them that you’ve published, and you can’t tell the seals from Iraq, so basically they were poring over pictures one by one and could not really count the seals accurately. Is that a fair way to characterize it?
Dan Morris: That’s right. And in fact, it’s even a little worse than that. If you imagine flying over the Alaskan coast and pointing a camera down to the ground. It’s not just that seals are hard to find. There are 99.99% of those images have nothing interesting in the middle. There just images of water and ice on something like two million image pairs to find something like a thousand seals.
Tom Temin: How did AI come into this? Because, first of all, there’ss pictures, as you say, that have nothing in them. But even in the pictures with seals, they’re pretty darn hard to spot from the air because they’re just sort of black blobs.
Dan Morris: That’s right. Well, one thing that NOAA has working for them is that they aren’t using just color images. They also captured thermal images, and seals are cold, but they are a lot warmer than ice, so the seals are much more visible in those thermal images. Really to see what’s going on, you need to look at both of the thermal images and the color images. But even in those thermal images, there’s lots of other things that pop out as being warmer than the ice, and so even in this thermal images, where you’d like a seal to appear as a nice bright dot compared to the cold ice, there’s lots and lots of bright dots and AI gave us an opportunity to let a computer look at all of those bright dots and the color images and try to help them figure out which ones were seals.
Tom Temin: Maybe the initial thing the computer could do would rule out all of the extraneous imagery that had nothing in it that would be of interest.
Dan Morris: That’s right. That is probably the most important thing we can do for them, because again, in this case, that’s 99.99% of the images. So although it’s potentially valuable to also go in, for example, classify which type of seal you’re looking at in a particular image, the real benefit and the real time saving comes from getting rid of all of those empty and uninteresting images.
Tom Temin: With respect to identifying what are finally, in the end seals. When I think of artificial intelligence, I think of software that is constantly evolving as it’s trained and learns. So tell us about the AI aspects of simply identifying dots from the wrong dots.
Dan Morris: That’s right. Just like with most AI and machine learning problems. The real secret is part algorithms, but more than that, it’s just having a really great data set to teach the machine what you wanted to recognize and what you don’t. So the first step in this project was to get all of those images from NOAA, which by itself was no small task because it’s about 20 terabytes of imagery, and get a really well organized table of which of these images have seals and where are they and which these don’t have seals? NOAA at this point, the point we kicked off this project, had already gone through and annotated all of the images from their 2016 flights. So we had this amazing resource of, here’s two million images, and here’s the couple thousand that have seals, and here’s exactly where in those images the seals are, and that’s exactly what you need to teach an AI model how to find the thing you want in new images. So that’s really where we started.
Tom Temin: And could this be applied to future samples of imagery where the scientists don’t have to spend all that time going through them one by one by hand?
Dan Morris: Exactly. That’s the idea. In fact, the idea is really two fold. We take all that data that was collected in 2016 and they had already put all that time in to labeling. And now hopefully we can train the model and then do two things. One is the next flight. When they come back with lots and lots of images, hopefully we can require much less human time to annotate all of those. But really where they want to go is even one step beyond that, which is, if you can run these AI models on the plane instead of back at home base. Then hopefully you could come back with only mostly interesting images and never even store all those extra images in the first place. Which not only gives you fewer images to deal with, but hopefully lets you move eventually to a paradigm where you can take many, many more flights on unmanned aircraft and really scale your ability to collect data, not just save people time which is really important, but scale your ability to collect it in the first place.
Tom Temin: You’re also working with scientists at NOAA that are examining sound waves beneath the surface of the ocean and using artificial intelligence there. Tell us about that part of the project.
Dan Morris: That’s right. We started this great project with the seals group at NOAA, and when it was going well, you know, one project leads to other, people know people and they introduced to another group at NOAA that’s using hydrophones, or underwater microphones, to survey both beluga whale populations and man made noise in the areas where belugas exist. And one of the many things they’re studying is how boat/vessel traffic, affect beluga behavior and beluga populations.
Tom Temin: That is to say, noise from man made sources interferes with the whales ability to communicate by echo sonar as they do, correct?
Dan Morris: That’s right. Or otherwise disturbs them or causes them to move from one area to another, exactly.
Tom Temin: So, is this a way to be able to count those whales and filter out the sounds that don’t count, but does it in some way., it can’t really help the whales navigate if there’s sound there because you can’t do anything about the sound being generated by, I guess outboard motors or whatever.
Dan Morris: Let’s say, both of these projects I would think of as scientific projects that are important for guiding policy about what areas we protect, what vessel traffic we allow, where, and it’s kind of a long road to get there. But ultimately the goal is, of course, to guide the policy that protects all of these animals.
Tom Temin: So, for example, I’m just making this up, if someday they discover that wherever powerboats are used of certain magnitude or horsepower, the beluga population should be X, but in these areas it’s X minus N, whereas where there are no sounds from man made sources, the beluga population is the expected X, and therefore you could decide whether to allow boats or what size of boats that kind of thing. Is that a good example?
Dan Morris: There are all kinds of potential policy implications and potential recovery effort, reintroduction effort implications. We very much trust our colleagues at NOAA for the scientific and policy sides of these questions. But there’s all kinds of implications of understanding critically endangered populations.
Tom Temin: But that would be a good hypothetical, not to say that they’re going to do that. but that’s the type of question you could ask?
Dan Morris: That’s right.
Tom Temin: The relationship with NOAA, you said that the AI for Earth program is part of a grant making. What’s the relationship? Is their contract with NOAA? Is there a grant from NOAA or to NOAA? How does that all work?
Dan Morris: Both of these products really fall under our technology development component of our program. We have a whole grant program also, but these are really part of our technology development component.
Tom Temin: So you have a contract with NOAA to do this?
Dan Morris: We don’t exactly have a contract. We have agreements in place that let us work with their data. But we for the most part of just working on a shared mission to protect wildlife.
Tom Temin: Dan Morris is principal scientist for the AI for Earth program at Microsoft. Thanks so much for joining me.
Dan Morris: Thank you.
Copyright © 2024 Federal News Network. All rights reserved. This website is not intended for users located within the European Economic Area.