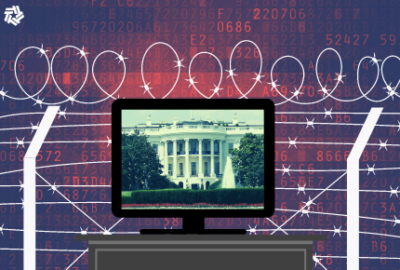
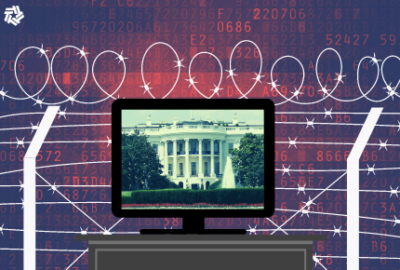
Hubbard Radio Washington DC, LLC. All rights reserved. This website is not intended for users located within the European Economic Area.
Artificial intelligence is steadily making its way into federal agency operations. A problem is that it can introduce unwanted biases.
Best listening experience is on Chrome, Firefox or Safari. Subscribe to Federal Drive’s daily audio interviews on Apple Podcasts or PodcastOne.
Artificial intelligence is steadily making its way into federal agency operations. It’s a type of software that can speed up decision-making, and grow more useful with more data. A problem is that if you’re not careful, the algorithms in AI software can introduce unwanted biases. And therefore produce skewed results. It’s a problem researchers at the National Institute of Standards and Technology have been working on. With more, the chief of staff of NIST’s information technology laboratory, Elham Tabassi, joined Federal Drive with Tom Temin.
Interview transcript:
Tom Temin: Mr. Tabassi, good to have you on.
Elham Tabassi: Thanks for having me.
Tom Temin: Let’s begin at the beginning here. And we hear a lot about bias in artificial intelligence. Define for us what it means.
Elham Tabassi: That’s actually a very good question and a question that researchers are working on this, and a question that we are trying to find an answer along with the community, and discuss this during the workshop that’s coming up in August. It’s often the case that we all use the same term meaning different things. We talk about it as if you know exactly what we’re talking about, and bias is one of those terms. The International Standards Organization, ISO, has a subcommittee working on standardization of bias, and they have a document that with collaborations of experts around the groups are trying to define bias. So one there isn’t a good definition for bias yet. What we have been doing at NIST is doing a literature survey trying to figure out how it has been defined by different experts, and we will discuss it further at the workshop. Our goal is to come up with a shared understanding of what bias is. I avoid the term definition and talk about the shared understanding of what bias is. The current draft of standards and the current sort of understanding of the community is going towards that bias is on in terms of disparities in error rates and performance for different populations, different devices or different environments. So one point I want to make here is what we call bias may be designed in. So if you have different error rates for different subpopulations, face recognition that you mentioned, that’s not a good bias and something that has to be mitigated. But sometimes, for example, for car insurance, it has been designed in a way that certain populations, younger people pay more insurance at a higher insurance rate than people in their 40s or 50s, and that is by design. So just the difference in error rate is not bias on intended behavior or performance of the system. It’s something that’s problematic and needs to be studied.
Tom Temin: Yeah, maybe a way to look at it is If a person’s brain had all of the data that the AI algorithm has, and that person was an expert and would come up with a particular solution, and there’s a variance between what that would be and what the AI comes up with — that could be a bias.
Elham Tabassi: Yes, it could be but then let’s not forget about human biases, and that is actually one source of bias in AI systems. The bias in AI system can creep in in different ways. They can creep into algorithm because AI systems learn to make decisions based on the training data, which can include biased human decisions or reflect historical or societal inequalities. Sometimes the bias creeps in because the data has been not the right representative of the whole population, the sampling was done that one group is over represented or underrepresented. Another source of bias can be in the design of the algorithm and in the modeling of that. So biases can creep in in different ways and sometimes the human biases exhibit itself into the algorithm, sometimes algorithm modeling and picked up some biases.
Tom Temin: But you could also get bias in AI systems that don’t involve human judgment or judgment about humans whatsoever. Say it could be a AI program running a process control system or producing parts in a factory, and you could still have results that skew beyond what you want over time because of a bias built in that’s of a technical nature. Would that be fair to say?
Elham Tabassi: Correct, yes. So if the training data set is biased or not representative of space of the whole possible input, then you have bias. One real research question is how to mitigate and unbias the data. Another one is that if during the algorithm biases if there’s anything during the design and building in a model, that it can be bias, that can introduce bias, the way the models are developed.
Tom Temin: So nevertheless, agencies have a need to introduce these algorithms and these programs into their operations and they’re doing so. What are some of the best practices for avoiding bias in the outcomes of your AI system?
Elham Tabassi: The research is still out there. This is one of those cutting edge research and we see a lot of good research and results coming out from AI experts every day. But really to mitigate bias, to measure bias and mitigate bias, the first step really is to understand what biases and that’s your first question. So unless we know what it is that we want to measure, and we have a consensus and understanding and agreement on what it is that we want to measure, which goes back to that shared understanding of bias or definition of bias, it’s hard to get into the measurement. So we are spending a little bit more time on getting everybody on the same page on understanding what bias is so we know what it is that we want to measure. Then we get into the next step of how to measure, which is the development of the metrics for understanding and examining and measuring bias in systems. And it can be measured biases in the data and the algorithm, so on so forth. Then it’s even after these two steps that we can talk about the best practices or the best way of mitigation of the bias. So we are still a bit early in understanding on how to measure because we don’t have a good grip on what it is that we want to measure.
Tom Temin: But in the meantime, I’ve heard of some agencies just simply using two or more algorithms to do the same calculation such that they be the biases in them can cancel one another out, or using multiple data sets that might have canceling biases in them just to make sure that at least there’s balance in there.
Elham Tabassi: Right. That’s one way, and that goes back to what we talked at the beginning of the call about having a poor representation. And you just talked about having two databases, so that can mitigate the problem of the skewed representation or sampling. Just like that, in the literature there are many, many definitions of the bias already. There’s also many different methods and guidance and recommendations on what to do, but what we are trying to do is come up with a set of agreeable and unified way on how to do these things thing and that is still cutting edge research.
Tom Temin: Got it. And in the meantime, NIST is planning a workshop on bias in artificial intelligence. Tell us when and where and what’s going to happen there.
Elham Tabassi: Right that workshop is going to be on August 18. It’s a whole day workshop. Our plan was to have a demo today but because it’s virtual workshop, we decided to just have it as one day. The workshop is one of the workshop in a series that NIST plans to organize and have in coming months. The fields of the workshop that they are organizing and planning is trying to get at the heart of what constitutes trustworthiness, what are the technical requirements, what they are and how to measure them. Bias is one of those technical requirements and we have a dedicated workshop on bias on August 18 where we want them to be a interactive discussions with the participants and we have a panel in the morning. The whole morning is dedicated to discussions of the data and the bias in data, and how the biases in data can contribute to the bias into whole AI system. We have a panel in the morning, kind of as a stage setting panel that kind of frame the discussion for the morning and then it will be breakout sessions. Then in the afternoon, the same format and discussion will be around biases in the algorithm and how those can make an AI system biased.
Tom Temin: Who should attend?
Elham Tabassi: The AI developers, the people that are actually building the AI systems, the AI users, the people that want to use AI system. Policy makers will have a better understanding of the issues in AI system and bias in AI systems. People that want to use it, either the developer or the user of technology, and policymakers.
Tom Temin: If you’re a program manager, or policymaker and your team is cooking up something with AI, you probably want to know what it is they’re cooking up in some detail, because you’re gonna have to answer for it eventually I suppose.
Elham Tabassi: That’s right. And if I didn’t emphasize it enough, of course at the research community because they are the one that we go to for innovation and solutions to the problem/
Tom Temin: Elham Tabassi is chief of staff of the information technology laboratory at the National Institute of Standards and Technology. Thanks so much for joining me.
Elham Tabassi: Thanks for having me.
Find out more information here.
Copyright © 2024 Federal News Network. All rights reserved. This website is not intended for users located within the European Economic Area.
Tom Temin is host of the Federal Drive and has been providing insight on federal technology and management issues for more than 30 years.
Follow @tteminWFED