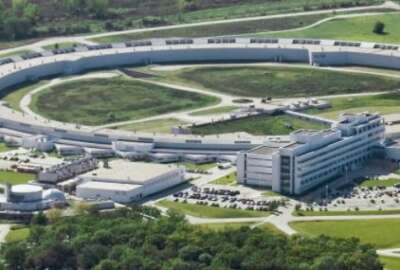
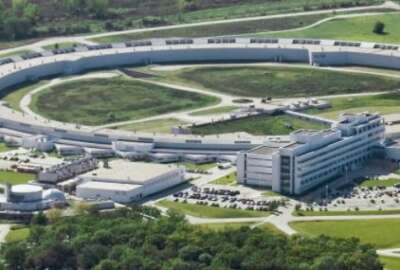
Hubbard Radio Washington DC, LLC. All rights reserved. This website is not intended for users located within the European Economic Area.
Nukes are expensive to operate. But researchers at the Argonne National Laboratory think they found a way to use artificial intelligence to drive down costs.
Best listening experience is on Chrome, Firefox or Safari. Subscribe to Federal Drive’s daily audio interviews on Apple Podcasts or PodcastOne.
If the nation is to have a so-called green future, many scientists and engineers believe more nuclear power generation will be part of the equation. Nukes are expensive to operate. But researchers at the Argonne National Laboratory think they found a way to use artificial intelligence to drive down costs. For more, the Federal Drive with Tom Temin turned to Argonne’s principal nuclear engineer, Roberto Ponciroli.
Interview transcript:
Tom Temin: There are lots of complications with operating nuclear power plants. Tell us what you’re looking at here with respect to artificial intelligence.
Roberto Ponciroli: The idea is that in the last few years, the nuclear industry is in terms of profitability not doing great. I mean, one way to 2018 studies, more than 1/3 of the units is either unprofitable or scheduled to close. So the point is that the Department of Energy is trying to identify solutions to improve profitability. So to help nuclear because as you said, nuclear better be part of the equation if we want a green future. And in particular, this is true for both for the current liberated units and the future units. So advanced nuclear reactors that might be deployed in in the current timeline in the power grid. So now, the point is that there are many ways you can try to pursue to reduce the capital costs and stuff. But currently, what we are focusing on is trying to reduce the operation and maintenance cost. So trying to limit the cost associated with the operation and the maintenance intervention. The idea here is trying to use the artificial intelligence, artificial AI algorithms to try to introduce elements of autonomy and automation in the operation of a nuclear unit. So to try to delegate to algorithms, lower level error prone repetitive tasks, that are currently taken care of by human operators, and to try to increase the situational awareness operator, what I’m trying to say is that low level things, so let’s have algorithms taking care of that. And let’s have human operators doing more interesting stuff, more important stuff, and having a more superficial role in the operation of a unit.
Tom Temin: What are some of the operations that could be applied here that would free up people from repetitive tasks and so on?
Roberto Ponciroli: As you said, when you have to operate a nuclear unit, the nuclear unit is an incredibly complicated system. As we said, there are hundreds, maybe 1000s of sensors of lights everywhere. And so it’s really based on teamwork. And it’s a very complicated job, you know, taking care of the for example, the performance of components, if a component is degrading, you got to be aware of that before taking an action or if you got to do some kind of power transits. Now there is the penetration of renewables, right? So that the demand is fluctuating, the renewables are fluctuating, and you gotta try to address this demand fluctuations. And you gotta try to adjust the power output of the unit accordingly. And that’s not straightforward. And as I said before, the operational nuclear unit is the result of teamwork, because there are operators, both technicians on site, and people in the main control room, they’ve got to talk together, and this kind of communication protocol. So now, it’s based on people talking to each other. But what if we can have algorithms helping these tests and favoring the coordination of all these aspects. And when I say aspects, I mean, the diagnostics, the control and the decision making.
Tom Temin: We are speaking with Roberto Ponciroli, principal nuclear engineer at Argonne National Laboratory. The information that you have put out on this talks about just simply having artificial intelligence, give a clue as to when a sensor might be ready to fail. And therefore you can replace it instead of having people test them and so forth. That seems like a pretty mundane operation. But what do you figure something like that could save a year in the operation of an average plant? If that’s even a calculable number?
Roberto Ponciroli: I can give you a number, you know, usually, because as we said, sensors, like every other component degrades. And so the sensors are subjected to periodic maintenance, right? Every one in a while you get up check that your senses are not biased or not off the accuracy and everything. And you know, there is a study that for me, it was it was really astonishing when I realized what they said in the study that when they checked the sensor, so they check all the sensors, but like 5% of the sensors need maintenance. The other 95% were just fine. So it’s a very repetitive, time consuming task. And while the thing is, we can have an algorithm telling us this sensor is off, you got to do something about these sensors, but all the other ones, they’re working just fine. So don’t waste time on that. And the reason why our research activity is focused on sensors is because we were thinking, in this world, we make decisions based on sensors. And if we have a very integrated the system, okay, when you have sensors telling you what’s going on, and you make a decision, and you take a control action, all this circuit is so integrated, if you commit a mistake at the very beginning, it kind of propagates very quickly, everywhere. So if your sensor gives you a wrong reading, your diagnostics are going to be off, your decision are going to be off and your control action can be really ineffective, or even worse, dangerous.
Tom Temin: Sure. And in the larger sense of knowing what operational level a reactor should have to respond to what is demanded of it on the grid, it seems like the AI could then go further beyond the perimeter of the plant, and look at weather patterns, wind and sun patterns, especially if you’re trying to fill in with very unreliable sources, like solar and wind, which is nevertheless policy for so many grid operators, that the AI could look at a lot of factors in the environment, to say it’s likely we’re going to need more power in seven hours, because there’s a cloud coming or whatever the case might be.
Roberto Ponciroli: That’s so true. Because the moment there’s another project, I mean, will they not were basically, we are trying to optimize a base capacity of the units. So what I’m saying is that I know the pattern of the sun, the pattern of the wind, and the predictions for future demand. So given though we would like to exploit as much as we can, wind and solar and sun power. So what is the optimal installed capacity. So that’s true if you have to build a new unit from scratch. But it’s also true, as you said, if you already have a unit, and I got to determine the best course of action in the next few hours in the next few days. Because as everybody knows, nuclear, it’s a kind of slow dynamics, right? So if you change power, then you can not like go up and down like a roller coaster. Okay, you got to stay there for a bit. So you got to think in advance what you’re going to do, and what’s the optimal decision, and definitely all this data, all the pattern recognition, research, it’s just so helpful to optimize the operation of the unit in the next few hours or a few days.
Tom Temin: Now, the Argonne National Laboratory has this knowledge, is there a way to get it promoted, say to the nuclear industry, and to those even the larger community of people that are thinking about what the future of the grid will be, and the need for the nation’s growing power requirements over the next years and decades?
Roberto Ponciroli: Yeah, totally. I mean, our national laboratories are currently pursuing a lot of research in the sensor in our division. In our group, we’ve been working for years on control, operation, diagnostics, optimization and stuff. And definitely we have a lot of contracts with utilities and the Department of Energy. And that’s a very cool part because we are working on real problems that we ask the utilities, hey, what’s your problem? And we’re trying to intervene and to share our know how, in our experience, to tackle this everyday problem that now in the recent days, and year in time, you know, they are getting more and more important.
Tom Temin: Sure, we’re going to charge those Tesla’s somehow and can’t do it on the grid we’ve got if there’s too many more of them. And just a final question, in the larger sense of, let’s say, the nation and policy and things come together, and that there is a movement to actually install new reactors. Could the process of siting them and proving the efficacy of the locations and so forth, which sometimes takes decades. And that’s why they never happen. Could that be improved? You think with AI at some point?
Roberto Ponciroli: Well, in terms of policy, I mean, it’s not really my area of expertise. So I’m not sure I can express the opinion. What I can tell you is that this architecture that we’ve been working on, that we’re trying to develop, but basically, it’s going to be really helpful for advanced reactor concepts. In particular, with respect to my the project I’m talking about here. It’s about the advanced and advanced reactor coupled with storage, you know, the battery basically. And so, the more the system is complex, the more you’re going to need an autonomous operation because the sequence of you know, tasks is, you know, is complicated and large and everything so, what I’m trying to say here is that this approach is going to be so beneficial for advanced reactors and definitely is going to help deploying this new technology.
Tom Temin: Roberto Ponciroli is the principal nuclear engineer at Argonne National Laboratory.
Copyright © 2024 Federal News Network. All rights reserved. This website is not intended for users located within the European Economic Area.
Tom Temin is host of the Federal Drive and has been providing insight on federal technology and management issues for more than 30 years.
Follow @tteminWFED