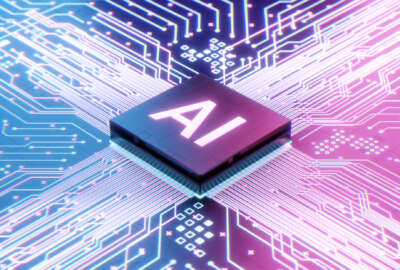
NASA computing and data science people are collaborating to find mission-related applications for artificial intelligence. For their work, they recently received an...
Best listening experience is on Chrome, Firefox or Safari. Subscribe to Federal Drive’s daily audio interviews on Apple Podcasts or PodcastOne.
NASA computing and data science people are collaborating to find mission-related applications for artificial intelligence. For their work, they recently received an award from ATARC, the Advanced Technology Academic Research Center. To hear more about their work, the Federal Drive with Tom Temin spoke with NASA’s David Na and Nikunj Oza.
Interview transcript:
Tom Temin
So you guys collaborated on artificial intelligence and machine learning transformational work there at NASA, tell us what this project was all about. David?David Na
One of the biggest pushes from the digital transformation effort is to open up and include more AI and machine learning capabilities for the greater NASA community. You know, this is a growing industry, there’s always new advancements. And we’re trying our best to remain on the frontier, our best to provide all the tools people need to do those types of research to do those types of, you know, machine learning models that you want to make on your specific data. And, you know, there but there are specific tools that they need specific computing infrastructure that they need to make that possible. And digital transformation has been making a huge effort to make that easier, better and much more streamlined for any NASA employee.Tom Temin
And Mr. Oza. What are some of the types of applications you can envision for AI and machine learning at NASA Ames? Maybe brief word on the on the focus of NASA Ames for those that don’t know.Nikunj Oza
So yeah, so NASA Ames itself has a pretty broad range of work that it does. So there’s work going on, for example, in life science, there’s work going on things like the heat shields for space vehicles to ensure that on reentry, it does not get too hot. There’s certainly significant work in aeronautics things like management of airspace, they are researching new approaches to allow the airspace to be better managed, as we have more and more air traffic not only from commercial vehicles, but from new entrants, like UAVs. So really, AI has quite a broad range of applications within all of these pretty much most of these systems, the engineered systems that we produce, and also natural systems that we study, such as the Earth and space through all the various sensors that we have, they produce a significant amount of data. And oftentimes, what we want to do is sort of reverse engineer those data, so to speak, I mean, we have some understanding of the processes that generate these data. But the data can really tell us what’s actually going on, that may be beyond what we really know is happening. So the data kind of can be like a source of truth as it were. And so we really want tools, you know, for everybody, you know, along the lines of David said, like, so many people are doing this, we really want tools to be able to allow people to really understand the processes that generated those data. Of course, just understanding that it’s not good enough, we do want to be able to act upon what it is that we learn. And so certainly, to a great extent, we would do so but we would also want artificial intelligence there to act on us. Because for example, when we’re, let’s say, on the Moon, or on Mars, we cannot necessarily depend on humans to do all of the acting, we really prefer in many cases to have an intelligent systems like a Mars rover that does the acting.Tom Temin
Right, because we know we can’t rely on the Martians either, for that matter. There is an underlying assumption here, though, so many agencies talk about applying AI and ML to the routine tasks, the business-oriented tasks, the processing of paperwork, that type of thing for their initial foray into AI, it sounds like you have the goal of applying it to mission critical, essential work of the agency, which is science and research and exploration. Is that a fair way to characterize it?Nikunj Oza
Certainly for like the work that I lead, as leader of the data sciences group, it is, as you’ve said, it’s very much the, what you would think of as the NASA mission and really trying to help directly with that, but certainly, the other kind of business processes. I mean, you know, NASA, just like other agencies, and companies have to go through those processes. They have substantial irritations in the process of going through them. And so we would like to see how AI can help with those. And let us focus more on kind of the core mission rather than all these side things that we find irritating.Tom Temin
Right, that we used to have secretaries do, we’re speaking with an Nikunj Oza. He’s data sciences group lead at NASA Ames Research Center. And with David Na, the foundation’s lead for the data sciences team at NASA. And David, there’s a cloud component to this, which seems to always come up as an enabling technology for AI and ML. Tell us more there.David Na
The cloud is a growing technology that basically allows you to scale your workload on not just your personal machine, but a group of personal, a group of machines that you technically don’t have access to, but through the cloud, you’re able to access and scale your workload to match whatever boundary or whatever capability you want. And that’s what’s amazing about the cloud is because we were at this point where you were limited by, you know, how much space was on the hyper the supercomputing machine at a specific research center, and you had to wait in a line to get your process running on that queue. But nowadays, you know, if your workload is transferable, which nowadays most workloads are, you can start that machine, that same job, you would have been running on a high performance computing machine on the cloud, right online from your local computer.Tom Temin
So finally, we’ve gotten past timesharing, it took 75 years, but here we are, I’d say, all right, and any early work that is producing results, I mean, given the range of possibilities that NASA could begin with for AI, where did you start? And what have you seen so far happen?David Na
Nikunj made a good point. NASA in general, we are mission focused here, we do have our different types of machine learning applications, but we’re mission focused. So the cloud by itself as well will be mission focused. One of the cooler projects I’ve seen worked is some stuff produced on the ISS, the International Space Station, we’ve had some prototypes for models that can detect wear and tear on astronauts’ spacesuits, we know we’re also working in collaboration with these cloud environments to make more hardware identification, tracking of, you know, specific hardware on ISS as well as additional parts to the spacesuit. And again, I want to make sure it’s also specified how we are being responsible with how we do this, how we conduct our AI and machine learning research, it’s never done without a human expert, looking over someone’s shoulder to make sure that all the data is being procured. And the mission is the focus for whatever is being done.Tom Temin
With the launch and reentry of the STS rocket, Orion capsule, this is a repeat, NASA has done this before back in the 1960s. Is there any thought, or is it practical to go back and maybe resurrect some of that data that could be reanalyzed with the tools we have nowadays?Nikunj Oza
I feel like it’s something that is well worth doing. Partially because without doing it, we don’t really know what are in the data. In fact, maybe they may not have been quite as much data generated back then, as there are now but so far all the data science projects that I’ve been involved in, have yielded surprise, or surprises pretty early on in the process. I mean, at the very least unexpected artifacts in the data where we see OK, some data transformation step gotten messed up or some sensor is not behaving the way it should. But in some cases, we did get unexpected insights into let’s say, unexpected relationships between components, like we thought they were operating independently, when in fact, they were not. So you know, I really see data science as almost like shining a flashlight into a dark room. So I think what what you say is well worth doing.Tom Temin
And given the number of projects, I imagine you must have a lot of incoming requests for I want an AI this or I want to ML that, I’m putting it crudely, how do you prioritize? And how do you make your decisions on what you’re going to do next?Nikunj Oza
Certainly, to a great extent we go based on programmatic alignment. So for example, within my group, we already have a significant emphasis on aviation safety for commercial aviation. So obviously additions to that will be much easier for us to take on because in some cases, there may not even be redundant work, and certainly life critical work, like what to mention, you know, where to say things like space suits, certainly, those would naturally get a higher priority as well. One thing I have found doesn’t quite answer your question, but I have had a few people come to me later, they said that they at one point earlier had an idea for a project, but felt like they did not have sufficient data to call us in and implement machine learning. That’s kind of a misconception. You know, yes, deep learning, which is very popular, does require a ludicrous amount of training data. But most of the machine learning methods actually do not. And so in fact, we do have machine learning algorithms that we’ve developed in house and certainly there are many others that have been developed elsewhere, where we have worked with tens or hundreds of data points, and have been able to get significant insight. So the amount of data that you have really should not be a barrier to start investigating the use of machine learning.Tom Temin
And David, let me ask you a final question. Are you also as part of this project, maybe training acolytes so that you can spread this kind of approach so that others in NASA can parallel your work?David Na
Absolutely. One of the biggest efforts of the digital transformation whole effort around AI and machine learning is to make it more accessible, removing the veil for people to see that it’s not like your data is just as applicable in machine learning at least some models, at least some applications as anyone else’s. And at the worst case, you’re experimenting with other people who might have run into the same problem you have and you guys are collaborating together. This whole collaboration environment. This whole opening up of this science sphere for this type of research is something that we’re heavily focused on with training, workforce development and collaboration.
Copyright © 2025 Federal News Network. All rights reserved. This website is not intended for users located within the European Economic Area.
Tom Temin is host of the Federal Drive and has been providing insight on federal technology and management issues for more than 30 years.
Follow @tteminWFED