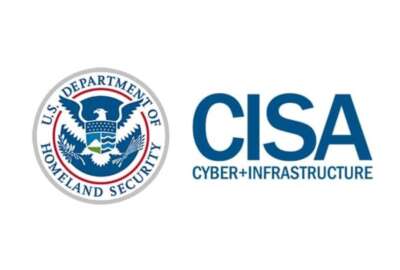
Hubbard Radio Washington DC, LLC. All rights reserved. This website is not intended for users located within the European Economic Area.
Hubbard Radio Washington DC, LLC. All rights reserved. This website is not intended for users located within the European Economic Area.
This content is provided by Jeskell Artificial intelligence (AI) has moved front and center as a consideration for federal agencies as they seek to enhance cust...
This content is provided by Jeskell
Artificial intelligence (AI) has moved front and center as a consideration for federal agencies as they seek to enhance customer experience, improve mission delivery, and modernize their organizations.
Use cases for artificial intelligence are everywhere. A few examples:
● Natural language processing. Machine Learning can help tackle some of the hardest problems in natural language processing including sentiment analysis, entity relationship extraction, lexical semantics, translation, and speech recognition. Whether it be improving stakeholder engagement, compliance review of documents, or domain specific research and analysis, every federal organization can benefit from reviewing how NLP can be applied to their mission set.
● Computer and machine vision. These systems translate digital images and video from their raw format into refined information which is more useful to computer systems or human users. Applications of computer vision include, but are not limited to, object classification, incident detection, automated inspection, and navigation.
● Predictive analytics. Machine learning techniques can enhance human decision-making and existing analytical frameworks by using historical data to provide inferences and recommendations about current information. Applications of predictive analytics include but are not limited to fraud detection, stakeholder management, risk management, predictive maintenance, and performance analysis.
The AI promise and challenges.
If the story of human advancement in the 20th century can be summarized as the pursuit to automate tasks requiring mundane, repetitive, physical exertion, then the story of 21st century may be described as the pursuit of automation of tasks requiring mundane, repetitive, mental exertion. This statement is far too early to validate, but research and application of artificial intelligence has so far been promising. AI can be described as the ability to represent a human decision process in a form that can be implemented by a computer against a given data set. AI is a tool to improve the efficiency of your employees, simplify engagement with your stakeholders, and increase the speed at which your organization can respond to changes in your operational environment.
For example, many agencies are responsible for reviewing and adjudicating cases, applications, or proposals in various forms. AI can provide employees refined information regarding compliance rules, risk analysis, and/or fraud detection. It can provide feedback to users at specific stages in any review process of possible errors, omissions, or requirements. Overall, this increases the efficiency, and in turn, the speed of the overall process.
Arguably the biggest field in AI is machine learning (ML). ML is the process of creating algorithms that can increase accuracy by iterating over data without needing to be explicitly programmed. Deep learning is a subset of machine learning which utilizes neural networks which are an interpretation of how the human brain processes information. Over time these approaches have been refined, computational power has become cheaper, and the amount of digitized information has grown exponentially. These advances have caused a tipping point that creates new possibilities for agencies to apply machine learning and artificial intelligence against a variety of problem sets.
While these possibilities are within reach, there are still challenges to effectively implementing machine learning. The first, and possibly most challenging issue, is the scarcity of personnel with AI and ML skills. Secondly, due to the scale of the data sets and the computational power required, it has caused organizations to rethink how they design computational systems to meet the demands of these workloads. As a result of these challenges, organizations are facing long development timelines before achieving the promised value from implementing these approaches.
Successfully overcoming these challenges requires the consideration of a number of factors. One consideration is how datacenter infrastructure is optimized to support AI and ML. IBM has developed a platform, PowerAI, which focuses on this problem set to decrease the time required to achieve useful models by providing easy-to-use bundles of the most popular open source machine learning frameworks on systems designed to provide exceptional performance running these workloads.
Building blocks
IBM POWER9
During the machine learning training phase the algorithm performs the same computation across multiple values in structured datasets. Doing these computations in parallel dramatically decreases the amount of time it takes to train a model. In order to achieve this parallelism, researchers have used Graphic Processing Units (GPUs) which while originally designed for computer graphics provide the capability to perform these parallel computations. When designing machine learning systems it is important to take into account how GPUs will be utilized and the unique performance characteristics they provide.
The bottle neck in these systems can quickly become transfer rates between the CPU and the GPU. The IBM POWER9 has been built from the ground-up for data-intensive workloads and provides state-of-the-art I/O subsystem technology. The ultra-high bandwidth CPU-GPU interconnect can deliver up to 4x faster training times. In addition to this incredible bandwidth benefit, the POWER9 supports advanced GPU/CPU interaction as well as memory sharing. In one experiment, a POWER9 accelerator coupled with an Nvidia GPU resolved in about 90 seconds a logistic regression classifier that took 70 minutes on a comparable popular cloud platform using TensorFlow.
IBM PowerAI
IBM PowerAI is a software bundle that runs on top of the IBM Power platform. The goal of PowerAI is to cut down the time that is required for AI system training, deliver enterprise-ready software distribution of open source frameworks for DL and AI, and simplifying the development experience. By creating this distribution, IBM has provided a mechanism for organizations to quickly get up to speed with optimized versions of these open source frameworks without needing to spend time implementing each individually, validating dependencies, and tuning performance. IBM provides support for all of these open source frameworks as a reliable service, in addition to the community support.
In addition to the core open source capabilities, IBM has provided components to further enhance the overall system. IBM Distributed Deep Learning provides a mechanism to scale the training over multiple nodes and up to 256 GPUs using optimized network communication to expedite the training process. In a distributed environment, updating a single parameter server can take nine seconds for even simple reduction operations utilizing fast network connections. IBM PowerAI DDL can perform the same operation in less than 100 ms by using a communication scheme that is optimized for the specific network topology.
Another enhancement provided in PowerAI is Large Model Support (LMS). The size of models can be limited by the amount of GPU memory that is currently available. LMS enables you to process and train larger models by utilizing main system memory coherently with GPU memory. LMS is enabled by the fast interconnect (NVLink) to accommodate such large models and fetch only the required working data to GPU memory.
PowerAI Vision
Case point: IBM’s PowerAI Vision suite. It’s designed to get organizations up and running quickly so that subject matter experts can spend their time focusing on their domain without requiring coding or deep learning expertise. By being accessible for business users this platform streamlines the workflow required to deploy an effective and powerful model. IBM’s Power AI Vision is a software library built from open source components, enhanced with IBM experience, and optimized for IBM’s Power AI hardware systems. With a few clicks, deep learning models can be trained to classify images or detect objects of importance.
Too often, barriers to entry into artificial intelligence are higher than they need to be. The turnkey approach to infrastructure lets an agency put AI to work faster, without worrying about the technical minutia of the underlying data science, building custom workflows, and relentlessly tuning hyperparameters.
Find a great partner
Having an integration partner who understands how to implement high performance, turn-key solutions will further enhance any agency’s time to value when it comes to implementing machine learning systems. Jeskell is such a partner to many federal agencies, and has a proud record of excellent past performance serving the federal market.
Jeskell, a long-term partner of IBM, has deep expertise in design, installation and configuration of POWER9 systems and the PowerAI software. POWER9 is an AI “secret sauce” – it simply can’t be matched by x86 systems for speed and availability. Jeskell’s engineers work with their customers to ensure the cluster delivered addresses all the customer’s unique operational requirements and constraints. Jeskell’s implementation, training, and consulting services allow agencies to quickly deliver models regardless of the current machine learning expertise available to the organization.
The company is bringing its experience to artificial intelligence projects at both the Defense Department and civilian agencies such as the National Institute of Standards and Technology. Many clients are looking at AI to help with analyzing exploding volumes of video surveillance data. As they learn, the systems can more accurately and consistently pinpoint objects of interest or the presence of a specific threat.
Jeskell has designed and built POWER9 clusters that can also accelerate a variety of tasks to reduce the dependency on human operators to evaluate and categorize items in audio and signal intelligence. These systems continually improve the fidelity of the algorithms used to identify objects or patterns of interest.
In short, it all starts with IBM’s Power hardware purpose built to accelerate and scale AI workloads. Topped by a validated distribution of fully supported and enterprise ready open source AI frameworks. All delivered, installed, and configured by Jeskell, an experienced small business that’s IBM’s leading AI partner.
Steven Koppenhafer is System Architect at Jeskell Systems, LLC.
Copyright © 2024 Federal News Network. All rights reserved. This website is not intended for users located within the European Economic Area.