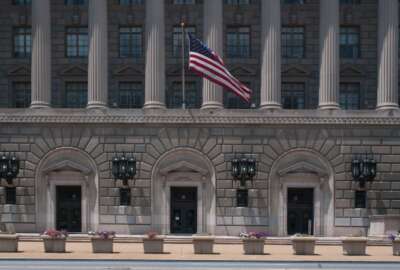
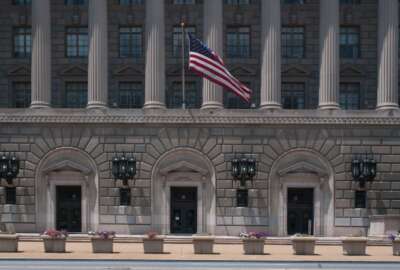
Everyone knows data is the essential element in improving government operations, understanding trends in the world, and solving big problems. Yet sometimes data can...
Everyone knows data is the essential element in improving government operations, understanding trends in the world, and solving big problems. Yet sometimes data can reveal too much, like people’s personal information. That is why data sets have to undergo what is known as de-identification. Now the National Institute of Standards and Technology (NIST) has updated crucial guidance on how to do this. For more, the Federal Drive with Tom Temin spoke with NIST Computer Scientist Simson Garfinkel.
Interview transcript:
Tom Temin Tell us exactly why the identification is required. Is it because of the application to which the data might be applied, or why do people need to do this?
Simson Garfinkel Well, I think we need to just step back a bit. Federal agencies operating under the Open Data Act are required to make data sets in their possession available to the public. Many federal data sets don’t have any privacy sensitive information in them. But some do and some have information that’s sensitive for businesses. So agencies that want to make those data sets available to the public, need to have some way of removing the information in those data sets that could damage privacy damage, proprietary interests while still providing value to data users. And that’s the topic that the draft publication, that was just closed for comment, that’s the point of that publication.
Tom Temin Right. And this is a reissue of some older guidance. So what has changed now? What caused NIST to decide that you need to get a new draft out there and get comments?
Simson Garfinkel Well, actually, it’s not a reissue of older guidance. The guidance was never issued. Back in 2016 a draft was published. And then due to a number of internal issues, that draft was never finalized. And so over the years, it’s been an effort to finish that draft and actually bring it across the finish line. And so that’s what this is about. Until this document is issued, it’s not guidance, it’s just a draft document.
Tom Temin Got it. But what was the issue the last time around? People overwhelm comments? Or you said it was an internal issue.
Simson Garfinkel The issue was that the individuals working on it, were working on other projects.
Tom Temin All right. And when you do de-identify, then it sounds like it’s just a matter of removing certain information from a database and leaving the rest. Or is it more complicated than that?
Simson Garfinkel Well, unfortunately, it’s a lot more complicated than that. Many years of experience have shown us that if you simply remove obvious information and release a data set, that information can be revealed through manipulations of the dataset or by linking the information that remains in the dataset with other datasets that are publicly available. In a previous NIST document, we detailed that. In this document, we reference that document and we also provide some more concrete guidance. I can give you an example if you would like.
Tom Temin Yeah, please do.
Simson Garfinkel Right. So one of the famous examples is that in the 1990s there was a request from the New York City Taxi and License Commission, for a list of all the taxi paths. And that was released under their Freedom of Information Act that their equivalent of it. And the taxi medallion numbers had been transformed, so they weren’t obvious. And the start and end locations of every taxi drive was left in the dataset. So one of the first things that was done, was that people realized that the transformation for the taxi medallion numbers could be backed out.. It was a hash then to sequence into an alphanumeric code, but it was possible to take all possible taxi medallion numbers, hash them and match them up. And then other people noticed that if you look at the start location and at the end location, there were some locations that were unique for individuals. So it was possible, for example, to see people who were starting a taxi ride at a strip club and ending their taxi ride at a residence, and then you could infer that there was some relationship between the person who lived at that residence and the the strip club.
Tom Temin We’re speaking with Simson Garfinkel. He’s a computer scientist at the National Institute of Standards and Technology. So the guidance that you are hoping to publish, now that you have the comments in the draft. When it becomes published, will it be designed for technical people to know how de-identify thoroughly? Or will it be for, maybe, higher level people, not higher level, but people that are less concerned with the technical details that need to understand that their data that they release is safe?
Simson Garfinkel But this is a guidance for government agencies, and the private industry is welcome to look at it. And it’s meant for both data practitioners, as well as for policy people in the privacy office. And it’s also meant for regulators to consider that it’s general principles for de-identified government datasets. So the the previous publication I wanted to reference was, NISTIR 8053 De-Identification of Personal Information. And that was published in October 2015. It’s still current and it has many examples of information datasets that were released, that were thought to be properly de-identified, that were not properly de-identified.
Tom Temin And there’s a term differential privacy that comes into this and that is somehow different from de-identification. Can you explain that concept?
Simson Garfinkel So De-identification is a general principle, it’s a goal. Some people use it as a specific set of mechanisms. And the NISTIR talks about the differences and the SP800-188 discusses differential privacy as an approach that might be used for de-identification. Differential privacy is a mathematical definition of privacy that has been developed since 2006. It was used in the 2020 census to release data sets of the number of people living on each block of the United States. And it’s going to be used for other data products from the 2020 census. And the idea of differential privacy is to carefully control the privacy loss that individuals suffer when their private data is used to create a public statistical product. Differential privacy is one approach that you could use de- dentification. There are other approaches. Unfortunately, there’s less mathematical or formal basis for those other approaches. People who use them hope that they work. They’re much more, say, aspirational than differential privacy. But there’s no way to know if they actually are working. And that’s one of the problems that we have with them.
Tom Temin Right. So then just to get back to de-identification there, the objective is such that when the dataset is released and has been de-identified, nobody can make correlations through some other means and reconstruct what was taken out, with respect to personally identifiable information.
Simson Garfinkel So that’s actually not true. Unfortunately, there’s no way to have an absolute guarantee of privacy or an absolute guarantee that there’s no risk. We can simply lower the amount of risk and we can lower the amount of privacy loss that individuals suffer. One of the reasons that we’ve had some challenges in getting this out, is that there’s a lot of disagreements in the data user community between people who are using old legacy techniques for de-identification where they believe that they could have an absolute assurance of safety and people who are up with the current mathematics, the current research, which shows that there is no way to be totally safe. Differential privacy forces you to confront that. And techniques that don’t use differential privacy are more sort of like, fire and hope. Like you use the approach. You think that it’s going to work, but there’s really no mathematical underlying basis that it will work. And that’s what leads to the sort of privacy problems that we documented in this, in this 8053.
Tom Temin OK. So people should read both. And you mentioned that the comments were closed, as we speak. If someone still wants to comment, will you take it in and have a look?
Simson Garfinkel I’m sure I’ll receive any comments that come in. Even if they come in after the window. We just can’t guarantee that for a significant comments that we’d be able to take them into account. But we’ll certainly look at them.
Copyright © 2024 Federal News Network. All rights reserved. This website is not intended for users located within the European Economic Area.
Tom Temin is host of the Federal Drive and has been providing insight on federal technology and management issues for more than 30 years.
Follow @tteminWFED