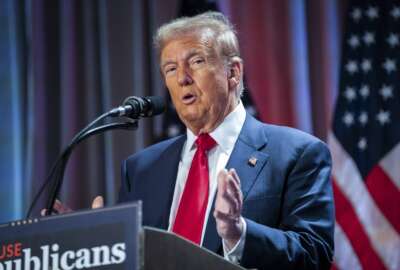
Federal News Network’s Tom Temin interviewed Elliot to better understand the impediments to taking AI to production levels, and the best practices for overcoming...
In nearly every discussion concerning federal IT modernization, artificial intelligence comes up as a central requirement. Experimentation with AI – examining algorithms or applying training data to them – is taking place across the government. Yet relatively few experiments, less than half, actually make their way into production environments.
According to Josh Elliot, a Vice President at Modzy™, a principal reason for the low adoption rate is technical. Agencies often have “rigid architectures and inconsistent, or even constrained, networking environments. Those are very difficult to simulate in a lab environment.”
Federal News Network’s Tom Temin interviewed Elliot to better understand the impediments to taking AI to production levels, and the best practices for overcoming them.
Elliot said that besides difficult environments, people issues also impede getting the most from AI work.
“Data scientists aren’t systems engineers, or software developers, and they don’t have the skills or expertise to design models that actually will scale in a production environment,” Elliot said. “And then, vice versa, software developers or engineers rarely have the machine learning background or expertise to design applications around some of the principles that are important to data scientists – like transparency, explainability, and accountability.”
That means the first requisite for AI success is making sure projects are backed by comprehensive teams that include not only the software and data science people, but also the program or line of business owners, and even senior agency management.
But you also need a more repeatable and disciplined approach to deploying AI, just as for success in any type of software. AI and machine learning algorithms often lack a window into how they work. Sometimes the algorithms produce variable results depending on the environment, rendering them untrusted or unreliable. Modzy addresses this concern with a model management framework. In it, AI teams can drive towards greater standardization and transparency.
Elliot adds that with better documentation – a “biography” of elements for each algorithm – coupled with an auditing and monitoring regime can help agencies move to a “ModelOps” a consistent, repeatable process for managing the lifecycle of machine learning models in production systems. Modzy’s platform, designed to move organizations from the experimental mode to ModelOps, provides “centralized AI management, for deployment, model orchestration, production, inference and monitoring, so that you can understand the performance of your AI and how it’s being used across the enterprise.”
Elliot added, “This combination of ModelOps with DataOps and DevOps tools and technologies, really is going to allow your organization to scale the use of AI. And that means shorter time to value, faster iteration, to fielding new AI capabilities,” all at lower risk.
Listen to the conversation for comprehensive information on how to gain greater traction and success with artificial intelligence in your agency.
Copyright © 2025 Federal News Network. All rights reserved. This website is not intended for users located within the European Economic Area.
Vice President and Head of Operations, Modzy
Host, The Federal Drive, Federal News Network
Vice President and Head of Operations, Modzy
Josh Elliot is a Vice President and the Head of Operations for Modzy where he focuses on driving growth, forging industry partnerships, and helping customers achieve their goals through the responsible adoption and use of emerging technology. Josh has a B.A. in finance and computer information systems from James Madison University, and is certified in the governance of enterprise information technology through ISACA.
Host, The Federal Drive, Federal News Network
Tom Temin has been the host of the Federal Drive since 2006 and has been reporting on technology markets for more than 30 years. Prior to joining Federal News Network, Tom was a long-serving editor-in-chief of Government Computer News and Washington Technology magazines. Tom also contributes a regular column on government information technology.