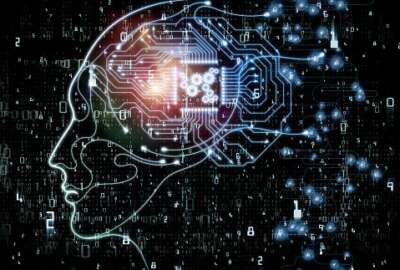
For all the talk in government about artificial intelligence, many of the AI tools in use by federal agencies today carry out a modest set of responsibilities.
Subscribe to Federal Drive’s daily audio interviews on Apple Podcasts or PodcastOne.
For all the talk in government about artificial intelligence, many of the AI tools in use by federal agencies today carry out a modest set of responsibilities.
While AI software can work on routine tasks around the clock with a high rate of accuracy, their use in federal offices has been limited to crunching numbers from databases and fielding basic customer service questions online through AI avatars, like the U.S. Customs and Immigration Service’s online assistant “Emma.”
However, Rep. Will Hurd (R-Texas), chairman of the House Oversight and Government Reform Committee’s Subcommittee on Information Technology, said he sees a larger role for AI in carrying out the business of government.
“It should make every interaction an individual has with the federal government take less time, cost less money and be more secure,” Hurd said Wednesday at a subcommittee hearing on the future of AI.
Within his own office, Hurd said caseworkers on his staff routinely hear from constituents about late veterans benefits or delayed benefit payments from the Social Security Administration.
“They’re speaking every day with people who are frustrated with how long it takes to resolve problems in the federal government. I believe with the adoption of AI, we can improve the response time, and in some cases, prevent these problems in the first place,” he said.
While AI has become a recent buzzword in the federal IT world, the technology has been around for decades. In the 1960s, the Defense Advanced Research Projects Agency helped stand up the technology and still plays a major role in the latest AI research.
John Everett, the deputy director of DARPA’s Information Innovation Office, told the subcommittee one of the biggest limitations of today’s machine learning systems is that they cannot explain how they’ve come up with a particular answer.
In light of this challenge, Everett said DARPA has begun looking into “explainable AI,” which maps out how AI software goes through its decision-making.
“The objective of the research is to say, ‘Tell me why you think is a certain kind of bird,’ and it will tell you, ‘Well, I think it’s got a red crest and a black stripe on the wing,’ and then it will show you that it’s actually looking at the right part of the image,” Everett said.
Another part of DARPA’s research focuses on building trust and reliability into some of its more ambitious AI projects, like an autonomous ship called Sea Hunter it’s been building with assistance from the Office of Naval Research.
“To ensure that it would operate safely within shipping lanes, it has to pass the commercial collision regulations. So we’re looking at ways to use mathematical techniques to verify that the software will behave as expected in a wide range of circumstances that it might encounter in the real world,” Everett said.
Rep. Paul Mitchell (R-Mich.) said part of the problem with AI is explaining its value to less tech-savvy sectors.
“There seems to be an innate fear of what AI is,” Mitchell said. “How do we, at the federal level, overcome, or get these levels of understanding among the population as a whole? Not the tech population — they all think it’s cool — it’s the other folks. What AI is, how it makes decisions, and why it’s of value to them.”
Jim Kurose, the assistant director of computer science, information science and engineering at the National Science Foundation, said that with AI tools, human operators still make all the executive decisions.
“There’s a long history of relying on computation to help in making decisions. I think the key phrase that you mentioned was ‘AI making decisions,’ and in the end, it needs to be people making decisions with AI software,” Kurose said.
While AI has come a long way in its development, Everett said machines still fall short of humans’ ability to learn and adapt to their surroundings.
“Right now, we’re building tools, and the popular press makes it seem as if these are going to become autonomous and think for themselves, but that is very far from the actual case of things. We know that people learn by having as few as one examples, and yet we need terabytes of data to get our systems to learn. We may look back on this time as the era of incredibly inefficient machine learning,” he said.
Douglas Maughn, the director of the Cybersecurity Division at the Department of Homeland Security’s Science & Technology Directorate, told lawmakers that the state and local first responders he builds tools for don’t care whether they get AI tools or not. Rather, he said, they just want what works.
“When we talk to operators in the field, and they’re looking for solutions, they don’t necessarily say, ‘I need an AI solution to my problem. They come to us and say, ‘I need a new widget, but it looks like this,’ and then our job is to go find the researchers at the universities or the companies. A lot of it ends up being how do they think about solving it, and do they think about solving it in an efficient manner that can take advantage of new technologies,” Maughn said.
Copyright © 2025 Federal News Network. All rights reserved. This website is not intended for users located within the European Economic Area.
Jory Heckman is a reporter at Federal News Network covering U.S. Postal Service, IRS, big data and technology issues.
Follow @jheckmanWFED