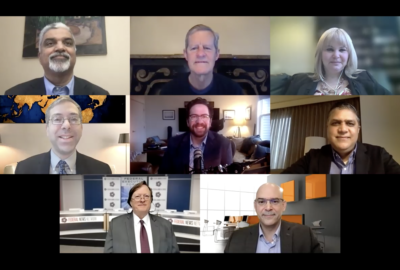
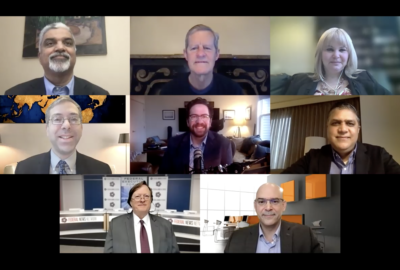
Contractors trying to develop artificial intelligence applications for the government face a challenge, namely a good data set for training the algorithms.
Best listening experience is on Chrome, Firefox or Safari. Subscribe to Federal Drive’s daily audio interviews on Apple Podcasts or PodcastOne.
Contractors trying to develop artificial intelligence applications for the government face a challenge, namely a good data set for training the algorithms. Now a big new federally-oriented data set is coming from an unlikely source. The Federal Drive with Tom Temin got the details from Bloomberg Government reporter Josh Axelrod.
Interview transcript:
Tom Temin: Well, first of all, where is this coming from? Let’s start there.
Josh Axelrod: So there’s an influential group at Stanford that has created a new data set that they call “pile of law”, it’s especially oriented towards legal and governmental contexts. Let me back up though, and tell you about foundation models, which they’re trying to make a better foundation model is basically, if you take like a row of Encyclopedia Britannica, and you try to ingest it all into a machine, and then use that machine to make decisions, learn something from the information you’ve provided it, a lot of foundation models, which is a term that comes from this Stanford group, and is now widely used across the industry. They ingest information from the public internet. So the internet, you know, I’m as much a fan of the internet as the next guy, but there’s a lot of crap on there. And foundation models have historically been trained using social media, Wikipedia, Reddit. So for example, yeah, if you’re trying to teach a model how humans speak, Facebook is littered with hate speech. And so that could encode bad grammar as well, which you can’t have in a model. So these researchers attempted to use a different type of data, they turned to casebooks, legal code, regulatory documents, again, things that are more suited to these legal and governmental contexts to do the work of natural language processing more effectively, which is really in use across the whole of government right now.
Tom Temin: Interesting. So in some ways, they are taking the approach that IBM did a number of years ago with a project they called Watson, where they would take all of the vetted material or peer reviewed material from a given domain and put that into a database, they had trouble selling it. I’m not sure how well it worked, it won Jeopardy. But that’s kind of what it sounds like here.
Josh Axelrod: That’s exactly what they’ve done is assemble this corpus of data. It’s actually 250-plus gigabytes. And it’s all open source. So programmers can come and look at that data, tinker with it, use it to build their own models. And again, it’s going to be better suited towards some of these regulatory contacts. There’s really five key areas where the government’s using AI and natural language processing, which this could really augment.
Tom Temin: And those areas are?
Josh Axelrod: Yeah, that would be enforcement, adjudication, regulatory research, public services, and internal management. Adjudications a really interesting one, the U.S. Patent and Trademark Office has been on the frontlines of that. I spoke with the director of emerging technologies, Jerry Ma. And again, he said that he sees the role of AI to augment the human expertise at that agency. So they use an algorithm to address what he calls the mail routing problem, just get applications in the hands of the right examiners, but lessen that burden on the human employees there. They also have this massive library of digital art, it’s only growing bigger every day. Sure, he was being a little cheeky. But he told me that if they don’t bring in an AI solution, that every working age adult would need to be patent examiners by the year 2050 just to address that ever growing library.
Tom Temin: Yes, I’ve heard Jerry Ma say that very thing. But the exponential growth in some of the sources that they need for prior art means they’ve got to have an automated type of intelligence solution. Because there’s just so much prior art out there in the world. Got it. All right. So this foundational model, the pile of law, which I can tell what everyone’s going to be calling it pretty soon, much as we love law and lawyers. But this is available to any agency that wants to use it from Stanford?
Josh Axelrod: Sure, absolutely. It’s open source. So people can can get in there and use it, people will typically take a foundation model and then use that corpus of data that’s already been catalogued, and something that’s called de-duped and cleaned and sorted. So really labor intensive process. And then they can use that to do some sort of natural language processing application. Something as simple as building a chatbot that can interact with the public, or as the U.S. Patent Office is doing use it to adjudicate claims more effectively.
Tom Temin: We are speaking with Bloomberg Government reporter Josh Axelrod. And as clean as a whistle as it might be, you know, the government is going to have questions about anything that dares to ingest because, and rightly so, because they want to make sure the algorithms that are deployed are unbiased and give results that, you know, in theory, if a human had the time would come up with, with a human knowledge. So is there a way for the government to vet this database and make sure that it really is a better source for training these five domain-related algorithms?
Josh Axelrod: Well, again, this is an open source data set. So it’s not like the government would kind of purely just map this into one of their applications. It’s a resource. And so the intention is to enhance the data that’s being used to create models. Anyone in the AI space will tell you, an AI model is only as good as its data is. That’s something that Jerry Ma told me himself. So people are aware that with bad data, you get biased and discriminatory results, sometimes just purely inadequate results, and you’re not going to churn out the results that you’re looking for. And so better data is key in this really, really nascent and promising market, to getting the results that you’re looking to get.
Tom Temin: And looking at this foundational model and the business behind it, Stanford University, did you discover whether it is possible to use a subset of it? Because you may not need the whole 250 gigabytes. And perhaps in a given application, you don’t want all 250 gigabytes, but you might need the 50 that are the most relevant?
Josh Axelrod: Absolutely, again, it’s this big corpus of data, you can pull from it, whatever you see fit. It was built with special filters for privacy and toxicity. Again, things that maybe social media companies are not going to prioritize as much, not something that you’re going to find in some of these foundation models that are scraping from the public internet, really just trying to ingest all the public content that’s out there. And I’ll give you an example of where this can be problematic. There’s a big AI contractor, ClearView AI, they’ve gotten into some hot water lately, but they’re used with law enforcement, with DHS, I demo-ed their product, and they took a picture of my face, they ran it through their platform, it’s really accurate. It’s like 99.9% accurate. They pull up, you know, 12 photos of my face. These are photos I didn’t even know existed on the internet. I never gave my consent to them being used. And so there’s this hotbed of privacy issues that emerges. This technology is both ubiquitous, and it’s nascent. And so we’re trying to work out some of those issues with privacy with bias with, you know, insufficiency of these models.
Tom Temin: Interesting. Yeah. I don’t dare google myself, because I know what’s out there. I don’t want to know what I don’t know. Right. So it’s just better to let a sleeping dog lie. All right. So then, are there developments that you’re aware of in other domains for foundation models, say, bioscience or any of the other strategic issues? The government is looking at public health, for example?
Josh Axelrod: Absolutely, you can use foundation models to do a whole range of things. So the government, you know, I told you about patent adjudication, that’s not the sexiest issue, but you can use AI to forecast hurricanes, you can use AI to help sort through drone surveillance. Again, this is something that’s being used, I had one sort of say, to his knowledge, he’s not aware of any agency that’s not using AI in some capacity, even if it’s as simple as transcribing meetings. So this is something that is ubiquitous across government agencies, and is only going to continue to grow.
Tom Temin: And by the way, you’ve done some research into what the AI market looks like in the federal government. What does it look like?
Josh Axelrod: Right, so according to data from our Bloomberg, government analysts-defined market for AI and machine learning, fiscal 2022, saw $1.4 billion in reported spending towards AI, that market first passed the billion dollar threshold in fiscal 2019. And it peaked in fiscal 2020 at $1.9 billion. Again, we don’t have all the figures in for this fiscal year. So that number is going to continue to grow. Just to put that in perspective, that’s a 225% surge from fiscal 2017 to 2021. Much more explosive than another emerging market cloud services.
Eric White: Josh Axelrod is a reporter with Bloomberg Government.
Copyright © 2024 Federal News Network. All rights reserved. This website is not intended for users located within the European Economic Area.
Tom Temin is host of the Federal Drive and has been providing insight on federal technology and management issues for more than 30 years.
Follow @tteminWFED