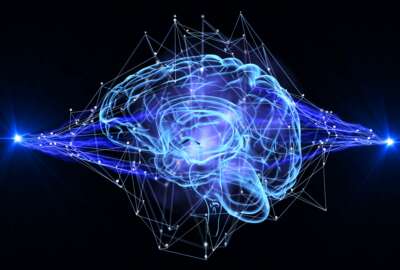
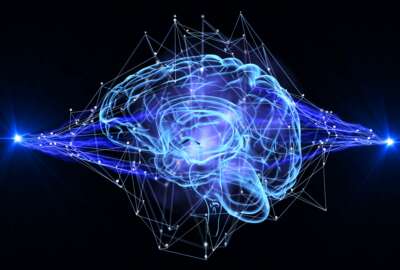
Chris Ritter, at the Idaho National Laboratory, said that while artificial intelligence is about getting computers to think like humans, machine learning is about...
Artificial intelligence and machine learning have emerged as important tools for modernizing systems and simplifying federal processes. Nevertheless, they require the right data for training the algorithms.
Humans train algorithms by using them and over time, algorithms learn via a “deep neural network.”
Chris Ritter, leader of the Digital and Software Engineering group at the Idaho National Laboratory, said that while the ultimate goal of artificial intelligence is to get a computer to think like humans or surpass humans in terms of predicting functionality, machine learning is about having pre-programmed devices, which can conduct analysis on their own. Scaling up general artificial intelligence, from something like a simple Google CAPTCHA form to operating a nuclear reactor, is what his office looks into.
“Where a lot of the existing research is, is in getting the data curated, and getting the data in a format that’s possible to get those scale-up advantages, and to apply machine learning to some of our complex problems in the energy domain,” Ritter said on Federal Monthly Insights – Artificial Intelligence and Data.
Aside from deep neural networks, which are a kind of “black box” not easily audited, Ritter said another kind of algorithm is called “explainable or transparent artificial intelligence.”
“What that means is, it’s mathematical. Right? So it’s completely auditable. And we can apply some penalize regression techniques to those areas, and you can make that a more novel technique,” he said on Federal Drive with Tom Temin. “And what a lot of people don’t think about is, if you have a ton of data – image recognition is a great example, right? Then DNN – these deep neural networks – are a great approach. But if you have less data, sometimes it’s better to apply a common statistical approach.”
In use cases such as life safety and critical safety systems, it’s important to be able to audit what the algorithm will do and why that is.
At the Idaho National Laboratory, Ritter engages in digital engineering which uses key tenets of modeling, building from a source of truth, and innovation – to name a few. The group has tried to change the way people work and have them produce data into buckets that engineers can already mine. Ritter said they’re trying this approach rather than seeing how they can make an algorithm smarter. Let’s make the humans change their pattern a little bit.
On the innovation front, he cited the Versatile Test Reactor project as an example. The reactor is being built to performing irradiation testing at higher neutron energy fluxes than what is currently available, and as a result could “help accelerate testing of advanced nuclear fuels, materials, instrumentation, and sensors,” according to the Energy Department. Ritter said a lot of university researchers were incorporated into the project, who bring novel AI techniques to the table.
To ensure digital engineering of these massive projects at the laboratory produce usable, real-world results, engineers build ontologies, or blueprints, for the data to curate it. Examples of data could be equipment lists, computer-aided design files, costs, schedule information, risks and data from plant operators, Ritter said. When these subsystems are generating so much more data than anyone can possibly look at in an hour, predictive maintenance can spot anomalies and raise a red flag.
“And so in other applications and other industries – we’re seeing predictive maintenance applied. And so we know that that technique is certainly possible, in the design side – being able to apply artificial intelligence during the design of an asset,” he said. “I think we are still in the early stages of that idea.”
Copyright © 2024 Federal News Network. All rights reserved. This website is not intended for users located within the European Economic Area.
Amelia Brust is a digital editor at Federal News Network.
Follow @abrustWFED