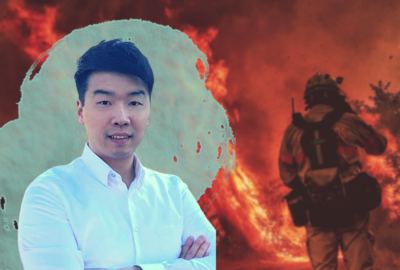
Ever since there have been building fires, firefighters have had to worry about the dangerous phenomenon known as a flashover.
Best listening experience is on Chrome, Firefox or Safari. Subscribe to Federal Drive’s daily audio interviews on Apple Podcasts or PodcastOne.
Ever since there have been building fires, firefighters have had to worry about the dangerous phenomenon known as a flashover. Now researchers at the National Institute of Standards and Technology have developed an artificial intelligence tool that promises to help in predicting flashover. For more details, NIST mechanical engineer Andy Tam spoke to Federal Drive with Tom Temin.
Interview transcript:
Tom Temin: Dr. Tam, good to have you on.
Andy Tam: Thanks, Tom. I’m glad to be here.
Tom Temin: And let’s talk about flashover itself. What exactly is it in the first place?
Andy Tam: This specific term might not be familiar with too many of us. So maybe let me take a minute or so to explain what flashover is and why it’s so dangerous. So flashover is an extreme fire event. When it occurs nearly all directly exposed combustible materials, like [a] sofa, mattress, wood cabinet, even carpeting in a compartment such as [a] living room, bedroom or even kitchen can be simultaneously ignited. And because of that, when everything is burning at once, the gas temperature within that compartment would increase dramatically within a very short period of time. And the temperature would typically exceed more than 100 degrees C, so in such a high-temperature environment, even when the firefighters are fully equipped the survivability will still be very low. And unfortunately, this kind of rapid fire progression, such as flashover has been the No. 2 cause for firefighter deaths and injuries. So we hope that if we can do something, then we can save many lives.
Tom Temin: And it’s hard to observe conditions that are leading to flashover. And so you have developed an algorithm or a predictive model. Tell us what it is you have fed into this model, tell us how it works.
Andy Tam: So our focus is to develop a model that can actually be used in real life, and we will find scenarios. The idea behind it is to use information from off the shelf fire protection devices, such as temperature reading from heat detectors within the building. However, there are two major challenges for this kind of realistic application. The first challenge is that there’s no perfect sensor or heat detectors that can withstand this kind of high temperature environment. And typically, heat detector would likely stop functioning without giving any reliables temperature signals at around 150 degrees C. Again, we have to bear in mind that the potential occurence of flashover usually happens when the upper layer gas temperature reaches about 600 degrees C. So this is a challenge that we have to overcome with. And the second challenge is that we actually do not have any information about the fire location, what item is being burned, or how large the fire is, and also quite importantly, we do not know any information about the opening status of the doors and windows for the buildings. However, we do believe that if our model is well trained, it should be able to recognize flashover based on the limited temperature information from the heat detector. Being said that, then the next challenge will be – we have to find a way to obtain the data.
Tom Temin: In other words, if the heat detector has a certain rise before it quits, and you extrapolated from that phenomenon, then that could give a clue that this is going to get really hot, and cause flashover.
Andy Tam: Right.
Tom Temin: All right. And I guess my question is, how many rooms and homes generally have heat detectors in the first place, though?
Andy Tam: So let me focus on another aspect of it, because each home will be different. But when we try to develop a machine learning based model , it s well known that we need a large amount of relevant data. Before our case, it means that we need to burn down a building structure many, many times. And if we think about it, carrying out hundreds or even thousands of this kind of large-scale experiment will be relatively difficult. And this because it’s going to be very time consuming and expensive. And also, there’s no guarantee that the data that we get is 100% useful. So in order to overcome this lack of data problem, we use what we call the learning by synthesis approach. And what that means is that we will carry out the full scale experiment virtually using a NIST fire model called CFAST. For this particular model development, we select a popular single-family building structure. And we conduct large number of numerical experiments with a wide range of fire conditions from burning a wood chair to all the way to burning a full size mattress. In our experiment, we also consider different opening doors and windows condition to try to mimic different fire events that happen in real life, such as a door being opened due to evacuation, and window breakage due to high temperature difference. But one thing that I would like to point out here is that we have carried out more than 20,000 different fire cases. But we have only about 5,000 cases with flashover that we can use to train our model. So if we were to physically conduct the experiment ourselves, it is going to be a very difficult job. But we were very happy that we have found a way to overcome that and obtain synthetic data for our model development.
Tom Temin: Yeah, I guess that’s better than burning down a whole neighborhood to check it out. We’re speaking with Dr. Andy Tam. He’s a mechanical engineer in the Fire Research Division at the National Institute of Standards and Technology. Alright, so the model, has been verified with real and simulated fire data. How does this work operationally? I’m a fire platoon. I’m going to a burning three bedroom raised ranch. How do I know that flashover could happen?
Andy Tam: So first of all, this model is still in proof of concept stage. However, we do anticipate these kind of questions. So when we trying to develop our models, one question that we will always try and ask ourselves is that, since our model is being developed based on synthetic temperature data, can it be trusted to be used in real fire scenarios. So in order to address this concern, we have tested our model against full scale experimental data obtained from the underwriter laboratories with the same building structure. One thing that I would like to emphasize here is that our model never sees any of these experimental data during the training process. So [it] is a very good reality check for our model, and see whether or not the model will actually work in real fire conditions. The results are very promising, and we have an overall accuracy of about 85%. However, theyre are still additional work that we have to do to improve the model performance. So in the following years, we will be conducting our own full-scale experiments with the integrations of our modeled into the fire alarm control unit, and we will test it against real fire.
Tom Temin: So you’re going to build some houses and burn them?
Andy Tam: Right, that’s gonna take time to build the house first.
Tom Temin: Yeah, but that sounds like the fun part of the whole experiment. And eventually, then, this would be an application that might exist on a radio or say a smart device that a firefighter would carry with him or her to a fire.
Andy Tam: Yeah, we envision that this model is going to be implemented in a building structure with wireless fire protection devices. In a fire scenario, we do believe that fire data such as temperature can be data by, let’s say, heat detectors. And this information can then be processed locally, to provide actionable information to our firefighters, such as when or where there will be a flashover in a building structure. We believe that if the firefighters can have this kind of information before they arrive to the fire scene, then they can plan ahead for the fire attack and also the search and rescue strategies. However, the tricky part is that the – mechanism to be used to transmit the data to the firefighter has not been decided yet. In general, the research work is still ongoing. But then the emphasis will be that the system itself has to be affordable, it cannot be in the order of thousands-dollars and so on. And also, it should only use existing commercial devices. And these because we believe that wireless technology is advancing so quickly. And we are afraid much adapted to it. For example, myself, I can control my thermostat far away from home. So we believe that reliable data transmission can be achieved in the near future. And this is the direction that our research is heading to and again, we hope that what we are doing in our group at NIST can help enhance the situational awareness and also the firefighting safety for our firefighters.
Tom Temin: And just out of curiosity, how did you as a mechanical engineer get into this firefighting and fire prevention deal?
Andy Tam: That’s a very good question. And this because if we look back, this problem is one of the major problems associated with fire deaths and injuries. I happen to have background and also did my research work using neural network. And then in order to address this concern or this problem in real time, the traditional methods might not be too applicable because doing this kind of simulation of the flight is going to be very time consuming. And the use of machine learning will help us to overcome this numerical bottleneck, meaning that we did a lot of training, getting all the data beforehand, and then we come up with a neural network model, then when we use it is just calling the neural network architecture or the mathematical formulations, then the usability will then be very easy.
Tom Temin: Dr. Andy Tam is a mechanical engineer in the Fire Research Division at the National Institute of Standards and Technology. Thanks so much for joining me.
Andy Tam: Thank you very much for having me.
Copyright © 2024 Federal News Network. All rights reserved. This website is not intended for users located within the European Economic Area.
Tom Temin is host of the Federal Drive and has been providing insight on federal technology and management issues for more than 30 years.
Follow @tteminWFED