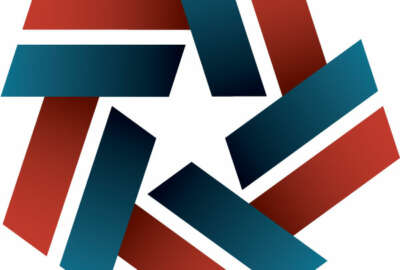
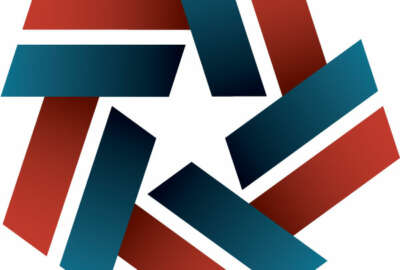
Intelligence agencies strive to implement AI and machine learning, but struggle to make data available for those machines to chew on.
Best listening experience is on Chrome, Firefox or Safari. Subscribe to Federal Drive’s daily audio interviews on Apple Podcasts or PodcastOne.
Like much of the rest of the government, the intelligence community is eager to take advantage of machine learning and artificial intelligence technologies to help make sense of its data. But first, the IC has a big problem to solve: making its information available to the machines. By some estimates, only about 2 percent of its vast data holdings are readily “discoverable.”
Efforts to boost that figure are part of the the latest iteration of the intelligence community’s multi-year effort to integrate its IT networks under the banner of ICITE. In what IC leaders have taken to calling the “second epoch” of ICITE, the focus is shifting from hardware and software to better data management.
“One of the near-term goals is to try and get a better handle on what data sets we actually have under our possession,” said Stephen Prosser, the intelligence community’s chief data officer. “It’s to do an inventory of all that data and then to put it in a catalog in order to improve our ability to discover data, so that we can then access it and use it. And for us, it’s a key component for how we approach artificial intelligence, machine learning, advanced analytics.”
But speaking at the Defense Intelligence Agency’s annual DODIIS conference in Omaha, Nebraska last week, IC leaders said there are already some early signs of progress. For one thing, each of the 17 agencies now has a chief data officer — something that wasn’t true a year ago.
And some of those agencies have projects underway to break their data apart from the stovepiped systems they’ve historically been associated with, moving them into flatter architectures that enable more sharing.
In one example, the National Geospatial Intelligence Agency has started to migrate some 20 petabytes of data from legacy systems stored at the Joint Warfare Analysis Center into Amazon S3 “buckets” within the intelligence community’s secure cloud.
Todd Myers, the automation lead at NGA said the project is about 90 percent complete.
“We’re going to have that data sitting in what I call ‘dumb buckets,’” he said. “Those buckets are going to be sitting there, available for us to leverage a clustered analytic compute environment that we have deployed. It runs the exact same things that Twitter and Apple and AirBnB use today to run massive data pipelines for correlated analytics. So we’re looking forward to being able to provide that to the community members, and really deliver extracted analytical information from full motion video, versus having analysts watching hours and hours of video for detections and so forth.”
The Navy is beginning to pursue a similar approach, said Dr. Ben Apple, the chief data officer for naval intelligence. He said the Navy’s analysts are starting to see data they’ve never had access to before, because it’s been locked in system-specific IT siloes.
“One of the things that’s doing is strengthening the analyst product. It’s helping the analysts move from a forensic model to a predictive model,” Apple said. “We can now start saying, ‘We think this will happen,’ because we can look at more data across more enclaves. The other thing that’s going to allow us to do is start to apply things like machine learning and artificial intelligence. These are technologies that require a great deal of data.”
Apple said he also expected a multi-fabric environment would reduce redundancies.
“Right now, if you look at the way things are structured, each enclave has its own database covering the same data,” he said. “By going to a multi-fabric environment, we start to break down those redundancies. It allows us to start identifying a true authoritative source for data, know where the data came from, what’s being done to it. It allows us to adopt, an enterprise-wide look at the data, instead of just covering the secret level or the top secret level or the unclassified level.”
But making data more widely available across IT systems and security classification levels within one intelligence agency is one thing. Doing it across all 17 turns out to be a much harder problem. That’s partly because each agency tends to have its own “lexicon” and categorization practices, and bridging those translation gaps is not easy.
An interagency working group is in the very early stages of trying to do just that.
Matt Cerroni, the chief data officer at the National Reconnaissance Office, said they began by trying to reach a common understanding of how to define the most basic of terms, like “dataset.”
“There was a tremendous amount of wailing and gnashing of teeth to decide, ‘What’s a dataset?’ We went around and around, and it wasn’t because we didn’t have the expertise – a lot of folks in the group are very plugged into data management,” Cerroni said. “We eventually went went from thinking about files, databases, directories, to a more philosophical view: the nature of information. What we backed into is the fact that a dataset is a collection of information that shares a particular attribute, but more importantly, it’s managed as a unit. So we’re making progress. It’s not very fast progress, but I think it’s very important.”
Busch, who now manages a DIA program called the Machine-Assisted Analysis Rapid-Repository System, claims collaboration is now the IC’s default position.
“The challenge of that is it forces us to rethink governance, because it’s not just authoritative versus less or authoritative,” he said. “It’s how do we present that information with an understanding that this is or isn’t assessed information. It’s either the stuff that’s had eyes on and is ready to go or it’s collected data that was machine generated and we’re not sure. This is a big challenge for us … the data has to be normalized, and 70 percent of the effort is data management. It has to be absolutely clean for you to go to AI. Your data can’t be throwing errors all the time.”
Copyright © 2025 Federal News Network. All rights reserved. This website is not intended for users located within the European Economic Area.
Jared Serbu is deputy editor of Federal News Network and reports on the Defense Department’s contracting, legislative, workforce and IT issues.
Follow @jserbuWFED