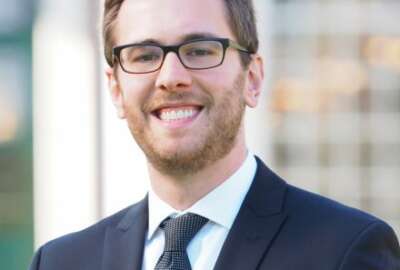
Federal agencies are embracing the idea of artificial intelligence, and in test cases, adopting machine learning has cut down on some of the tedious aspects of ...
The phrase “artificial intelligence” can stir up a lot of panic at some federal agencies, and can give rise to the idea of intelligent machines putting some employees out of work.
However, some federal agencies are embracing the idea of artificial intelligence, and in those test cases, adopting machine learning comes down to a few key strategies like starting small and managing expectations.
While AI isn’t a panacea for every big-data problem in government, agency leaders say they see value in using machine learning to handle the most tedious aspects of handling data, which frees up human operators to address more mission-critical issues.
“Artificial intelligence is an imperative. It’s not something that’s nice to have, or something that we should consider at some point,” Teresa Smetzer, the director of digital futures at the Central Intelligence Agency said Tuesday during an event sponsored by Partnership for Public Service and the IBM Center for the Business of Government. “We have an enormous exponential growth in the amount of data, the variety of data, the velocity of data, and our nation’s security really depends on our ability to quickly understand what data we have, what it means and how we’re going to use it.”
While still in its early stages, artificial intelligence has received lots of buy-in from the private sector and the academic world. But Rep. Pete Olson (R-Texas), a co-founder of the Artificial Intelligence Caucus on Capitol Hill, said the conversation around AI has not yet addressed the implications for lawmakers and the federal government.
“AI has the ability to provide lawmakers like me with up-to-date information, leading to better-informed decisions. And since AI never, ever forgets, its constant review of the effectiveness of policy gives lawmakers and government officials the opportunity to be proactive and address issues as they first crop up, and not wait to deal with them years and years later, when the problems get much, much, much bigger,” Olson said.
The key to going forward with new developments with AI, Olson said, includes protecting the privacy of individuals’ personal information in databases and educating the workforce to view artificial intelligence as a tool, and not as a competitor.
Mallory Barg Bulman, the vice president of research and evaluation at the Partnership for Public Service, said the rise of AI comes at a time when agencies face new technology-driven challenges, but haven’t received new funds or manpower to address them.
“We’re at a time in government where we’re not able to do more, with more,” Bulman said. “We’re really trying to look for that Option C. What is that other option? What is the way to do things differently to achieve critical outcomes?”
From a national security perspective, Smetzer said the CIA’s goal is to reach a stage where the intelligence community doesn’t just react to events, but also anticipates them. But to get there, the agency first has to make sense of the troves of incoming data it receives around the clock.
As the director of digital futures, Smetzer works closely with the private sector and universities to learn more about the cutting-edge uses of machine learning.
“We’re trying to leverage really the investment, which has grown three or four times over the last few years,” she said.
While artificial intelligence has proven value in a number of case studies, Claude Yusti, a federal cognitive solutions leader at IBM, cautioned viewing machine learning as the end-all-be-all solution for federal IT.
“No one sets out to do AI projects. That’s not the ambition,” Yusti said. “The ambition is, people have problems to solve, and a lot of times they’ve been stymied in terms of how far they can get with a solution. And the question is, what is the difference that AI brings to the equation that makes problems go away better, more effectively?”
In the case of the CIA, selling AI as the solution to technology challenges has meant taking an incremental approach.
“Start small with incubation, do proofs of concept, evaluate multiple technologies [and] multiple approaches. Learn from that and then expand on that. That’s the approach we’ve taken,” Smetzer said. “We have the advantage that we made a strategic investment four or five years ago into a cloud computing environment … but we still have a lot of work to do when it comes to the data and the expertise, and really solving our mission-use cases and problems.”
While AI does have national security implications, it can also be used by civilian federal agencies to reduce workers’ time on more tedious tasks. That has largely been the story at the Bureau of Labor Statistics, which used AI to compile data on workplace injuries.
For years, BLS employees have had to manually sort incoming descriptions of each injury case, including the names of occupations and industries.
“But as you can imagine, there’s millions of these data points, and so to figure out how to classify them was typically a human process that took a lot of time,” said William Wiatrowski, the acting BLS commissioner.
In one year alone, BLS saw more than 2,000 different job titles for a position that could generally be described as a janitor or cleaner. While making sense of all those titles would be a tedious task for a BLS employee, AI has reduced that burden on the bureau’s workers.
“Traditionally, we would have staff that would review that data by hand, and would determine that they belonged in Occupation Code X, which is the janitor and cleaner. That’s something that we can now use machine learning to improve the consistency,” Wiatrowski said.
In order to build momentum for machine learning at federal workplaces, Richard Ikeda, the director of the National Institutes of Health’s Office of Research Information Systems, agreed that the theme of starting small is the way to go.
“At NIH, there’s the enterprise-level IT systems, which would be an expensive place to basically integrate an AI system. But you can start with the institutes and centers themselves, where they have an issue that they need to tackle that takes a lot of time. And they have a little more flexibility than the enterprise does, and they can experiment and start small with a problem, and then finding out if it’s successful or not and move it to the enterprise,” he said.
Copyright © 2024 Federal News Network. All rights reserved. This website is not intended for users located within the European Economic Area.
Jory Heckman is a reporter at Federal News Network covering U.S. Postal Service, IRS, big data and technology issues.
Follow @jheckmanWFED