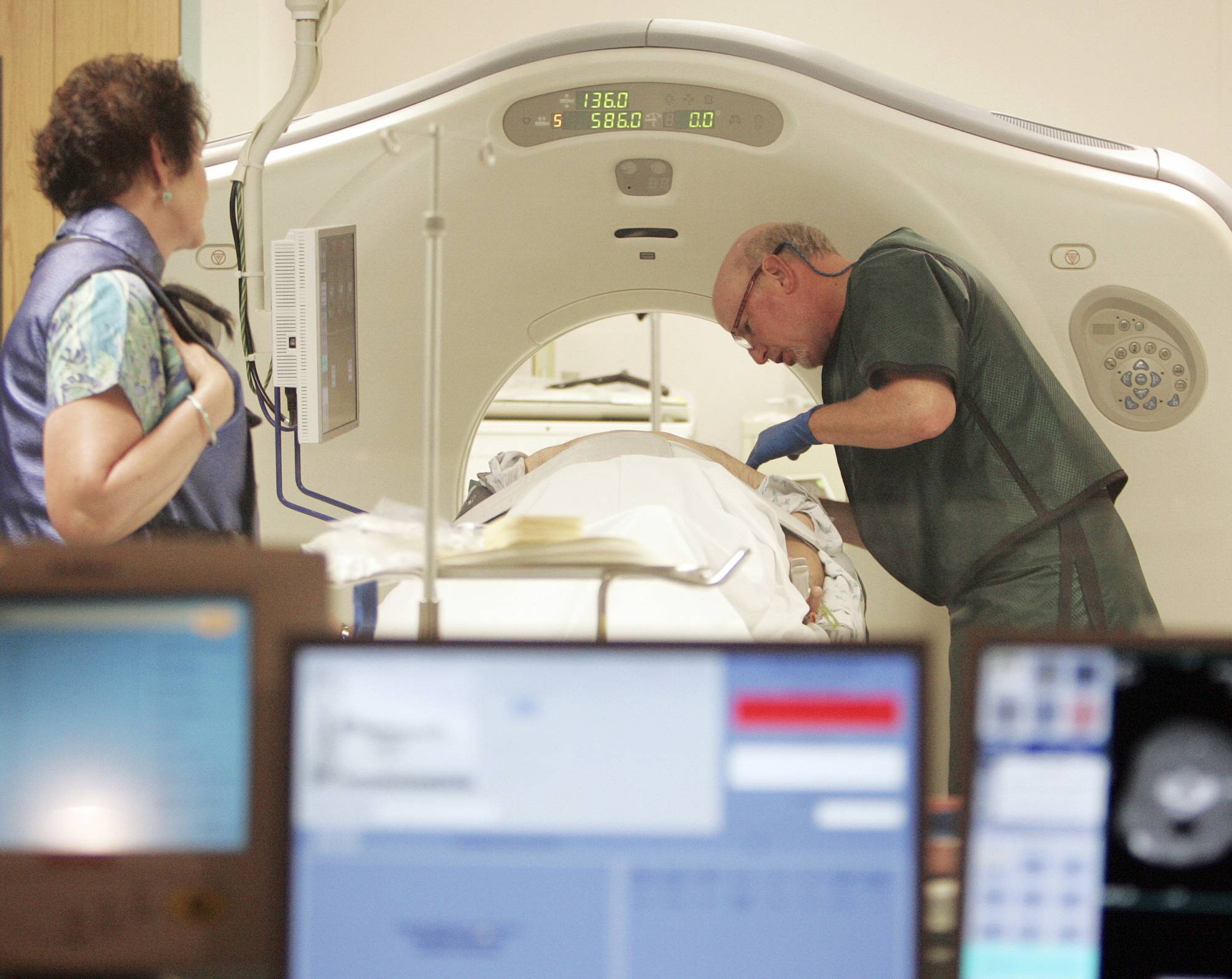
Hubbard Radio Washington DC, LLC. All rights reserved. This website is not intended for users located within the European Economic Area.
Hubbard Radio Washington DC, LLC. All rights reserved. This website is not intended for users located within the European Economic Area.
The work of our next guest has spanned 40 years and helped save lives. For that work at the NIH, he's a finalist for the Paul Volcker Career Achievement Awar...
The work of our next guest has spanned 40 years and helped save lives. For that work at the National Institutes of Health, he’s a finalist for the Paul Volcker Career Achievement Award from the Partnership for Public Service. Federal Drive with Tom Temin spoke with Dr. Rocky Feuer, the Chief of the Statistical Research and Applications Branch at the National Cancer Institute.
Interview Transcript:
Rocky Feuer So I founded and I currently lead a consortium of simulation modelers, I’ll explain what that is. Actually, for the last 23 years, we have over 200 investigators at over 30 academic institutions, and it’s called the Cancer Intervention and Surveillance Modeling Network, or CISNET for short. And it has really fundamentally changed how U.S. cancer screening guidelines are developed. So we’ve helped to support the US Preventative Services Task Force, that’s an independent panel of experts in evidence based medicine sponsored by ARC, to set and revise screening recommendations for long breast, colorectal and prostate cancer. So, for example, we help support the task force in a recent draft recommendation to start breast cancer screening at age 40 instead of 50, and a final recommendation for colorectal cancer screenings to start at age 45 instead of 50. And so you have the task force insisting that to thank if your doctor tells you to get a colonoscopy five years earlier than you would have otherwise, and it is really a good thing.
Rocky Feuer Before CISNET screening recommendations were based on what was called evidence reviews. These are systematic reviews of all known studies, but direct evidence from studies wouldn’t be sufficient to distinguish all the relative benefits and harms of a very large number of possible screening regimens. That’s age to start screening, age to stop screening, how often you should be screened. And that sometimes comes to hundreds of different combinations. You couldn’t do studies on all of those combinations. So population decision modeling, we simulate millions of people’s individual lives. We simulate the year they were born and what risk factors they were exposed to. For example, men born in the 1920s, many of whom fought in World War Two when they handed out Cigarettes’ to the GI’s, had the highest smoking rates of any generation of Americans. So they had higher lung cancer screening rates. And then if at what age did cancer start to develop in somebody’s body when the cancer would have caused symptoms and been diagnosed in the absence of screening, what type of treatment they would get and what age they would die of either their cancer or some other cause. And then over this, we superimpose many different screening schedules on their lives to see how things might have turned out differently. These are kind of called counterfactual situations. What if you didn’t have screening? What if you had screening that started at 40? What at 50? What if you had it every year? What would if you had it every other year? And then we accumulate all these results and determine the set of screening schedules that produce the most benefit in terms of lowering death rates and the fewest harms, for example, false positive screening results per number of screens conducted.
Rocky Feuer So when I started this consortium, I knew that simulation models contently be very valuable in this area, but they really had a serious credibility issue in some circles. Independently developed simulation models taking on the same problem often resulted in radically different results that were just very difficult to reconcile because there were so many complex and subtle differences between the models. So people felt, Ok, you get whatever result you want, and this greatly hurt the credibility that these models. So in CISNET we took a different approach. It’s a collaborative group of modelers for each cancer site with multiple independent models for each cancer site, but then they work together and tackle the same problems in a very systematic way. They share common inputs and produce a common set of outputs, and they get to understand each other’s models. So when the results are similar, it brings real credibility to the results. And when they differ, the reasons for these differences can be systematically evaluated. So this approach greatly improved the credibility of this type of modeling. And it’s not only become a critical tool in developing new screening guidelines, but are also used in other ways, for example, to understand the contribution of past advances in prevention, screening and treatment to national cancer trends and to project cancer rates into the future as a function of the uptake of some of the newest advances. And then importantly, studying the sources of health disparities in cancer rates and what might be done to reduce them. So this consortium has really, yeah, changed the game a little bit in terms of screening guidelines and other ways to evaluate at the population level what’s occurring and why it’s occurring and to project into the future.
Eric White So using this technique of modeling, I’m just curious on what went into the models themselves. Was it data that you all gathered from certain cancer sites or are numerous amounts of cancer sites and then you were able to replicate the results and that’s what you’re saying added to the credibility because it was showing that, Ok, yes, if we do X, then Y happens every time. Sort of deal.
Rocky Feuer Yes. Well, first of all, if the models got the same answers then we feel that way. But we use every possible data source, population based cancer registry, and I could talk a little bit more about that, is the backbone of the research. But we use national surveys of screening rates and smoking rates. We have something called a smoking history generator. We use national surveys from 1965 to the present to reproduce by birth cohorts smoking histories of individuals when they started, when they stopped, how many cigarettes’, and that’s an input into the models. We use screening studies because they kind of what’s called dip into the preclinical cancer and we could see how fast cancers are growing before they become symptomatic and how many people. We also use autopsy studies, there’s a number of studies where people die of other causes and then they might like biopsy their colon very closely and see how many of these people have polyps as pre-cancerous lesions, how many of colorectal cancer that’s been done for prostate cancer and other cancers. So we use every possible data source and then we calibrate the models based on that. And then in the end run, maybe there’s a new trial that occurs and we use the models to see if we could predict what the trial showed or if we could predict national trends and rates, and then we could decompose those rates. So we look on and on and on for all the different data sources. And what the models do is sort of synthesize all the data and then the comparative modeling, because people could take different approaches and get different results. But when they come together or if they don’t come together because we’re working together closely, we could understand the differences between the models.
Eric White Gotcha. Ok. And those discoveries that you talked about lowering the recommendations for screenings of colon cancer, things of that nature, have there been any population based discoveries that you all have made through these modeling systems?
Rocky Feuer So, yes, for just for example, in lung cancer screening, we use a criteria for if you’re eligible for lung cancer screening, we don’t screen everybody. We want to screen mostly fairly heavy smokers because because and we use a criteria called tac years. How many years to just smoke? Times how many packs a day you smoked? And African-American individuals tend to have similar pack years to white individuals, but they tend to start a little bit later in life. They don’t initiate at the same time, and their cessation is usually a little bit older. So they might have the same pack years, but it’s shifted to an older age. And we know from different studies that if you accumulate those pack years at a little bit at an older age, even though you have the same pack years as somebody else, you have a higher risk of lung cancer. And the lung cancer screening recommendations didn’t take that into account. So it created a health disparity. So what the new round of lung cancer screening recommendations, they lowered the threshold for being eligible for lung cancer screening to fewer pack years to accommodate, in general, more people. But especially African-American individuals who might have the same pack years as somebody else, but have a higher risk because they accumulated those pack years at a higher age. So that’s an example of a very careful study of the population based data and then how it translates into something like screening recommendations.
Eric White You all factored in advancements in treatments and screening procedures. I’m wondering what you think of advancements in screening technologies or the data accumulation technologies. Do you foresee a future where you’re having even more factors coming in that you’re able to make the model even more predictive and more accurate?
Rocky Feuer Well, yeah, Let me just talk a little bit about our population based cancer registries and how that data has radically improved over time. So cancer registries, that part of the program I work on correlates the population based cancer registry. Cancer registries collect data on every cancer that occurred and defined geographic region. It’s usually a state and really form the backbone of population based cancer statistics. And when I first started at the National Cancer Institute in 1987, our registries covered only about 10% of the U.S. population. And today, the National Cancer Institute in the Centers for Disease Control collectively have registries covering the entire U.S. population.
Copyright © 2024 Federal News Network. All rights reserved. This website is not intended for users located within the European Economic Area.
Tom Temin is host of the Federal Drive and has been providing insight on federal technology and management issues for more than 30 years.
Follow @tteminWFED