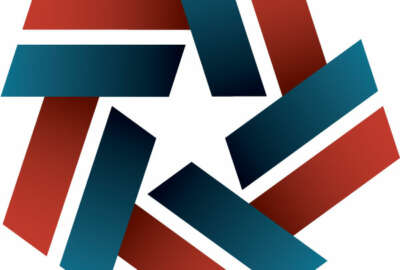
Hubbard Radio Washington DC, LLC. All rights reserved. This website is not intended for users located within the European Economic Area.
Hubbard Radio Washington DC, LLC. All rights reserved. This website is not intended for users located within the European Economic Area.
That's what Georgetown University's Center for Security and Emerging Technology set out to discover. Research fellow Tim Hwang joined Federal Drive with Tom Tem...
Best listening experience is on Chrome, Firefox or Safari. Subscribe to Federal Drive’s daily audio interviews on Apple Podcasts or PodcastOne.
A lot of corporate research effort goes into artificial intelligence and machine learning. But do the top tech companies’ investments coincide with national priorities for competitiveness and scientific leadership? That’s what Georgetown University’s Center for Security and Emerging Technology set out to discover. Research fellow Tim Hwang joined Federal Drive with Tom Temin for the findings.
Interview transcripts:
Tom Temin: Mr. Hwang, good to have you on.
Tim Hwang: Thanks for having me on the show Tom.
Tom Temin: So you set out to find out first of all, what the leading tech companies – Apple, Google, Facebook, IBM and a couple of others – actually put their money where their mouth is, so to speak, when it comes to artificial intelligence. And how were you able to find that out?
Tim Hwang: So one of the most interesting things that we see is that people are always talking about AI this, AI that, and we will think about AI as kind of this really broad, vague category. And then one of the things we’re really interested in exploring in this paper was specifically what are these labs researching in the field of AI? And the way we got to that was actually looking at their research publications, right, what their labs are putting out into the world, and using that as a way of inferring what their research agendas are.
Tom Temin: So this was a broad search of millions and millions of documents out there, because there is a lot published on this, isn’t there?
Tim Hwang: Yeah, exactly. And I think this is one of the big sort of things that we want to learn a lot about is, obviously there’s a lot of research happening in the field of AI, are the companies researching everything in that universe, or only specific things, right, and really kind of understanding what the delta is between what they do and what the sort of field as a whole is.
Tom Temin: And you look at the aggregate of these companies, and found out that the top topic for them to put their money into and the research into is robotics and grasping. Grasping I get given some of the companies here, but the human to machine robotics and so forth.
Sure. As in a hand grasping, right, yeah, exactly.
Tom Temin: And modeling of 3D shapes, and image enhancement and so forth, which you can definitely understand. But what does this tell you? I mean, the companies, by the way, were IBM, Microsoft, Facebook, Google, Apple, and Amazon, clearly the top leaders here, among them. And what does it say about them that that’s so important to them?
Tim Hwang: Yeah, what’s really interesting for me in doing this research is really two points. I think one of them is not surprisingly, it’s not like these companies are investing in AI for the good of human time, right, they are very much kind of focused on products, right, and doing R&D to eventually support their products and services. And so I think a lot of what you see is, in some ways, not too surprising, right. They’re investing in a lot of things that help them do image understanding and search, but then also that there is a big investment in robotics, because there’s ultimately going to be a lot of money in that space. So I think what we see is a research agenda that is ultimately very driven by their business models, no matter kind of what they say more broadly.
Tom Temin: And do you have a similar type of count in terms of where the energy is going for artificial intelligence and machine learning from the public sector?
Tim Hwang: Yeah, I think that’s right. And I think this is one of the things that the paper is really aiming to do is, when we think about AI and AI companies, we think about the six companies that you just mentioned, right, that are the focus of the paper. But there’s a lot of universities, as a lot of labs, there’s, in fact, a lot of other companies that are investing in different parts of the AI research field. And so I think, in some ways, our message to policymakers is to say, take a step back, don’t get sucked into the hype, let’s think about what we should be investing in as a country and trying to say, okay, are our partnerships pushing that forwards, right, versus just kind of getting drawn into the fact that these are kind of the cool hip AI companies.
Tom Temin: On the other hand, you could see where robotics and grasping modeling of 3D shapes, images, coloring, lightening, enhancing and so forth. These might be of interest to say, the Defense Department or other missions that the federal government does have. Correct?
Tim Hwang: Correct. Yeah. And I think I guess I’m a pragmatist about these sorts of things, right. I mean, I think the usefulness of these kinds of maps is the ability to say, okay here’s where our interests overlap, and here’s where we should be partnering. There’s so many AI initiatives out there that are just like, we’re going to put billions of dollars into AI, but they don’t really talk about where that money is going. And I think we need to get a lot more specific if we want effective national strategy in the space.
Tom Temin: And just a detailed question. One of the topics of importance to the corporate side is sparse matrices and representation. What is that exactly?
Tim Hwang: Sure, yeah. So this is getting a little bit into the weeds and kind of nerdy aspects of this. But a lot of what happens in AI is that you’re trying to teach a computer to understand a task. In the field, that’s known as a representation, right. What does the machine actually learn when you teach it that a cat looks like this, or a dog looks like that? So there’s a lot of work kind of in optimizing those algorithms. And that is actually what those topics refer to.
Tom Temin: Okay, we’re speaking with Tim Hwang, he’s a research fellow with the Center for Security and Emerging Technology at Georgetown University. It strikes me that knowing where corporate research is aimed, that would probably direct some of the grant making agencies, DARPA perhaps or National Science Foundation, in knowing where the dark spots might be, relatively speaking in artificial intelligence.
Tim Hwang: Right. I think that’s exactly right, what we learn from this kind of analysis is that private enterprise is selecting a certain kind of research agenda, right. And I think ultimately, what we need to ask is, okay, are those aligned with sort of the national interest? And to the extent that there is divergence, right, I think we want to think about these agencies, right, like DARPA, as gap pillars in the space to say, okay well maybe we need to find ways of encouraging more research on the places where the companies aren’t looking, because frankly, it isn’t very helpful for their product roadmap, for their services roadmap.
Tom Temin: And when the federal government funds research that can go directly into the public domain and software algorithms, for example, can go into open code, to what extent does corporate research end up in the public domain?
Tim Hwang: Well, yeah, I think this is one of the interesting things is we can only see what we see, right. And so our paper recognizes this, it’s a limitation like in that the only research that we know, that they’re focused on, are the things that they publish publicly, right. And that really is at the discretion of these companies. So I think that there are a lot of follow on benefits for federal funding and public funding, that you really don’t get totally relying on these corporate labs to kind of drive your AI national strategy.
Tom Temin: I guess maybe one question is whether the results of what corporate labs do will be used internally, or whether it will be used by, say, their vendors to develop new robotic systems, for example, just to make an example of robotics and Amazon?
Tim Hwang: I think that’s exactly right. I think that one of the interesting things to think about is these companies, needless to say, are not representative of the sort of institutions in society at large. So one of the things I point out in the paper, right, is a lot of the research that these companies are investing in assume that you have unlimited access to data. Now, I don’t know, in a liberal democracy, you really might want an AI to work in a way that’s a lot more sort of privacy respecting, right, or is able to do what he can do without collecting so much data. The companies are never going to invest in that because they already have all this access to data. So there’s really kind of these basic things that may create divergences between sort of what we want to technology as a society and what these companies are really investing in.
Tom Temin: Interesting. So what is your basic conclusion then in this study. It’s not really a long report, it’s only 18 pages with some big charts.
Tim Hwang: Yeah, we want to keep it short and precise, because I think the point that we’re making is, in some ways, really intuitive, but we don’t really talk about it in think tank circles or policymaking circles very much, which is that we need to get a lot more granular and use these types of maps to guide sort of research strategy in the space. Because I think that identifying these gaps is a really key way of thinking about AI. And getting away from kind of the early days of discussion about this, which really was we just need to have the best AI, right, I think we’re now entering a period of time to talk about like, what exactly does that mean, right. And I think this kind of analysis is really key.
Tom Temin: And I wanted to ask you about one of the appendix charts, which is really interesting, top colleges and universities and counts of AI publications in dimensions, as of May 29 2020. First let me talk about the colleges, except for Carnegie Mellon University, they all seem to be Chinese. I mean, even Harbin Institute of Technology, most people never heard of the city of Harbin in China but I think it’s got 10 million Apple, it’s small for China, but it’s really big. So what is that telling us do you think?
Tim Hwang: Yeah. What it’s telling us is a couple things. I do think that there is a lot of research activity that’s going on that almost never makes it into the kind of mainstream press in the US. And that’s partially due to a language barrier. And I think in many ways, does kind of limit our ability to do good strategic analysis in the space, because I think even simple analyses, like the one that we did in the paper, do indicate that if we’re measuring progress in the field just by raw number of publications, and now there’s problems with that of course, but if we just use that metric, it does indicate that we really got to put the pedal to the metal here if we want to keep up from a national standpoint.
Tom Temin: And you cite a source called Dimensions. What is Dimensions?
Tim Hwang: Dimensions is a database of research publications, and it’s really kind of our source for the analysis that we’re doing here. And one of the reasons we like Dimensions is because it has this kind of more global coverage. It starts to help us get a little bit of a picture of, okay, what does the research landscape look like outside of the ones that we always hear about right here in the US?
Tom Temin: It looks to me like it’s a wake up call for the US academic field.
Tim Hwang: Yeah, I think that’s right. And and I think one subtlety here, and that there’s a separate paper that I did about this, is that I would sound a note of caution on measuring sort of how successful we are based on just like the total number of publications, anyone can throw a paper up online. And so I do think that one of the key things that we need to look into is thinking not just about sort of publication count, but also citations, not only who is producing the most research, but who’s producing the most influential research. And I do think a lot of these kind of what they call bibliometrics are going to be key as we sort of understand who is really kind of leading in this field. And to the point of the paper that we’ve been talking about, who’s leading in what? Is it robotics and grasping that we’re really leading in or are the Chinese ahead on certain subfields within AI? And that subtlety is really important.
Tom Temin: So basically, you’ve raised a bunch of fresh questions about AI here.
Tim Hwang: Yeah. There’s always more questions than answers. But hopefully I think this perspective on these issues is an important one to bring into the mix as we kind of think about where we are going as a country on on this technology.
Tom Temin: Tim Hwang is a research fellow for the Center for Security and Emerging Technology, that’s part of Georgetown University. Thanks so much for joining me.
Tim Hwang: Yeah, thanks for having me.
Copyright © 2024 Federal News Network. All rights reserved. This website is not intended for users located within the European Economic Area.
Tom Temin is host of the Federal Drive and has been providing insight on federal technology and management issues for more than 30 years.
Follow @tteminWFED