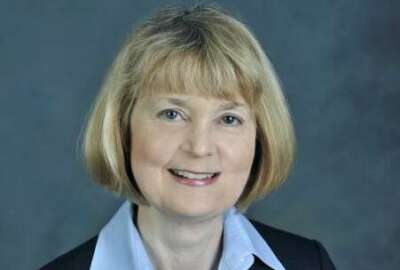
Hubbard Radio Washington DC, LLC. All rights reserved. This website is not intended for users located within the European Economic Area.
Hubbard Radio Washington DC, LLC. All rights reserved. This website is not intended for users located within the European Economic Area.
Gary Shiffman, who teaches security studies at Georgetown University, argues that the right screening applied at the right time would prevent the improper payme...
Best listening experience is on Chrome, Firefox or Safari. Subscribe to Federal Drive’s daily audio interviews on Apple Podcasts or PodcastOne.
The problem with finding out you’ve sent money to ineligible or deceased people is that it’s too late. Much of the money can be nonrecoverable. Gary Shiffman, who teaches security studies at Georgetown University, argues that the right screening applied at the right time would prevent the improper payments in programs like those under the CARES Act. He is founder of Giant Oak search technology, and joined Federal Drive with Tom Temin for more discussion.
Interview transcript:
Tom Temin: Dr. Shiffman, good to have you on.
Gary Shiffman: Tom, it’s great to be with you. Thank you.
Tom Temin: So your theory is that pre-screening before money goes out is something the government should be doing. And I guess my question is, how could that fit into the application process for funds, as we’ve seen it, say, in all of those loans that went out under the CARES Act?
Gary Shiffman: I would say it a little bit more forcefully. I think that not screening would be irresponsible. We’re in the middle of a crisis and we have to perform what I would call a dual mission. And that is we have to get in money out the door quickly to people who legitimately need the aid and assistance. And I’m totally in support of that, and we need to do that. But we also want to minimize fraud, waste and abuse. The dollars going out the door are just massive. And we know from history that when we send a lot of money out quickly, a lot of dollars are wasted. Following Hurricane Katrina and Rita, in retrospect, we now know that about 16% of the money, the $6.3 billion in relief that went out, went out to fraudulent claimants. So 16% of $6.3 billion is a lot of money. Right now, we’re talking about over $2.5 trillion has already been approved. And as we know, the House approved another $2.5 trillion bill and Senator [Mitch] McConnell and the Republicans in the Senate are going to propose about a trillion dollar additional bill. So we’re talking $2.5 trillion plus an additional between $1 trillion-$2.5 trillion. If we’re not screening ahead of time, think about the amount of money that could go to fraud, waste and abuse in fraudulent payments.
Tom Temin: And explain how screening might work in the context of artificial intelligence, because most screening right now is simply looking at applications. And I don’t know how much fact checking they really are able to do given the speeds required. So there’s an artificial intelligence and a speed element I would imagine, that would have to be brought into this.
Gary Shiffman: Sure. So eight years after the Katrina and Rita aid went out, we had as you recall, the financial crisis of 2008-2009. And again, the U.S. government stepped up and put out a lot of money. This time, we did screening ahead of time and the fraud rate went down from 16% to 0.2%. That difference was screening. The idea is when somebody applies for aid or assistance, you review the applicants, you review their business, you review the validity of their claims. The problem that we have today is one of speed. In 2008-09 we took two years to get the money out the door. So we know screening works. So the challenge isn’t is screening the right thing to do – screening’s clearly the right thing to do. The question is, as you’ve just posed it, how can technology help us today? So here’s how machine learning and artificial intelligence work: Machine learning is pattern recognition. That’s all it is. So you have patterns of behavior, you have patterns of fraudulent claims, you have patterns of legitimate claims. And you just train those in the model. You train models of the patterns that you’re looking for – the good patterns, the bad patterns – and then you run all of the applicants through these patterns. And what you are able to do is verify the goodness of almost all of the applicants. Because if you think about it, if you have an effective screening program in place, almost everybody applying for benefits is going to be a legitimate person or small business in the case of the PPP, applying for the benefit. You’re going to be able to verify and validate that quickly, get the money out the door and provide a massive disincentive to people who would want to cheat the system. And what I would really emphasize – and we can talk more about machine learning if you want me to drill down on that more – but it’s about putting a disincentive in place for the fraud. And we don’t have that today, and that’s why we’re seeing such spikes in fraudulent behavior.
Tom Temin: We’re speaking with Dr. Gary Shiffman. He is founder of Giant Oak search technology. And you began to answer one of the questions. Let me say, I’ve never applied for anything except a tax refund. So I have no record one way or the other. So if I made up a small business and applied for a loan that I wasn’t entitled to, you’re saying the goodness or badness could be verified. There’s no criminal record, there’s no prior fraud convictions. So I guess my question is, how difficult is it to detect, say the first-time fraudster that has no record?
Gary Shiffman: That’s a great question. So the first-time fraudster who has no record won’t show up in the databases of past convicted fraudsters. But that doesn’t mean that you can’t detect them. There’s a case study that I like of two small business owners who applied for PPP loans. One was approved, one was denied. Both were convicted felons. Both were running small businesses. One of them honestly said on his application that he was a convicted felon, he was denied. Another person lied about being a convicted felon was approved, got $2 million and spent the money on new cars and all kinds of fraudulent things. That’s the first case. We’re not even doing that right now. That’s the first case and that’s simple – that is, can you determine if somebody lied about their previous arrest records? So we have a fair amount of that going on where people are just able to simply lie and because of the speed at which we’re trying to get money out the door, we’re defaulting to approve the loan and then find that person later. So the deterrent effect is one of investigation after the fact and not screening upfront. And that was the problem from Katrina in 2005. That was a long time ago. It’s 15 years later, we need to be doing better than that. The second part of your question – a lot of the fraud in the PPP loans are coming from people who claim to have small businesses that don’t have small businesses. So this could be a first time fraudster who goes to the post office, opens up a P.O. box, creates some small business, goes to a financial institution, where they don’t currently have a banking relationship, opens up a bank account in the name of the business, puts the address of the business as the post office, and the post office boxes, the address says they have 10-15-20 employees and applies for a PPP loan in order to make payroll for the 15 employees who in reality are nonexistent employees. So even if that person’s never been a fraudster before, there are ways to determine that pattern of brand new business, 15 employees post office box, things like that. This is what I mean by machine learning can learn patterns like this, doesn’t mean that everybody applying for a loan under those conditions is fraudulent. But it means that that’s a popular scam, and machine learning can go through all of the legitimate loans very quickly and identify that less-than 1% that needs some sort of further due diligence.
Tom Temin: And let me ask you about another element in here and that is, I’m going to relate it to the continuous screening that is done now under the security clearance process where people are under what they call continuous evaluation. And that requires pulling in independent, private sector databases that are nevertheless available to the public domain, from the Experians, the TransUnions and so forth, and many, many others. Such that they can be alerted when someone gets into say deep credit card debt or some other issue that might indicate a security clearance issue. So does that analogy hold here that perhaps some of these programs could use those databases to enrich what they already know about people?
Gary Shiffman: Absolutely. There’s a very robust marketplace for data right now. I would argue that the publicly available data is really all you need. But if you want to augment it with some of the data that , I call it GLBA or Gramm-Leach-Bliley Act data. This is data that is privacy protected under the Gramm-Leach-Bliley Act that the credit bureau companies tend to have. So this is the credit header bureau in some of your credit data, which is also available under the Fair Credit Reporting Act. I don’t believe the Small Business Administration is using the Fair Credit Reporting Act data in evaluating PPP loans. But that’s a possibility and that would tell you if somebody has financial trouble. The point is that there are massive opportunities for looking at data for screening, but I think you have to balance privacy with the fraud mission. And so you might not want to use all of those data sets. And I think you can do the job of high speed, large scale screening, and continuous vetting, continuous monitoring. You can do all of that with purely publicly available data. And you can accomplish the mission, which is I just want to emphasize the mission isn’t to arrest and convict everybody who cheats. The mission is to put a deterrent in place. So those first time fraudsters don’t ever choose to commit fraud. And if you have a deterrent program in place, you’re going to be incredibly successful at this. And if you don’t have a deterrent program in place, and you’re relying on investigations three years from now to identify somebody who fraudulently obtained $200,000, I think we are going to largely fail.
Tom Temin: And just a final question, does this require on the part of the program owners in the government, some kind of a process to maybe devise or write up scenarios that they think could be used, and that those scenarios are loaded into your machine learning system. So it will know what patterns you wanted to watch for?
Gary Shiffman: The way to do this, and again, there’s an art to deploying machine learning that’s evolving now, because we’re in the early stages of this, but the first thing you want to do is you want to start with that human heuristic, that expert at detecting fraud. You interview them and you figure out well, what are the patterns that they’re expecting to see? And you start your modeling based upon the human knowledge. But then you want to open up the machine learning process to review data, as cases come in to learn additional dimensions of fraud that the human might be unaware of. And then of course, you want to let the machine do it a million times a second, and those are the steps and the processes to developing and deploying machine learning for screening and continuous setting.
Tom Temin: And I guess you would also have to be careful with how you deploy say characteristics such as zip code or location, because that could raise racial disparities and issues that are just going to get your program into trouble.
Gary Shiffman: Absolutely. What you want to look for are patterns of behavior and not demographics or identity categories. I write extensively about this. The problem we get into is when we attribute identity categories with behaviors, so we confuse – to be academic-y about it – to confuse correlation with causation. That’s the biggest mistake that government agencies and banks make today in the absence of machine learning. And my concern is that machine learning will just perpetuate those errors and those biases. We want to look for human behaviors that pose a threat to the system, and behaviors are blind to the category labels and we want to avoid category labels and just look at patterns of behavior. A fraudster is somebody who commits fraud, not somebody who lives in this zip code.
Tom Temin: All right, well, let’s hope they’re listening before they appropriate and send out this next, however many trillions. Dr. Gary Shiffman is founder of Giant Oak search technology. He also teaches artificial intelligence at Georgetown University. Thanks so much for joining me.
Gary Shiffman: Tom, enjoyed it. Thanks very much for all you do.
Tom Temin: We’ll post this interview at FederalNewsNetwork.com/FederalDrive. Hear the Federal Drive on demand. Subscribe at Apple Podcasts or Podcastone.
Copyright © 2024 Federal News Network. All rights reserved. This website is not intended for users located within the European Economic Area.
Tom Temin is host of the Federal Drive and has been providing insight on federal technology and management issues for more than 30 years.
Follow @tteminWFED